The advent of machine learning has significantly transformed the landscape of customer service, offering unparalleled opportunities for personalization. This technology not only saves employee time but also revolutionizes customer engagement by crafting experiences that are tailored to individual preferences and behaviors. By analyzing vast datasets, machine learning assists in creating realistic customer personas, predicting customer behaviors, and enhancing service quality. This results in reduced churn and increased conversion rates, ultimately leading to a more satisfying and efficient customer experience.
Key Takeaways
- Machine learning leverages large datasets to create personalized customer personas and predict behaviors for enhanced engagement.
- Personalization and predictive analytics in AI contribute to proactive customer service, reducing churn and boosting conversions.
- Generative AI models utilize customer data for tailored responses, with observability and prompt management ensuring controlled outputs.
- AI-powered tools such as chatbots and virtual assistants offer hyper-personalization in customer interactions, especially in retail and e-commerce.
- Responsible AI practices ensure precision and adaptability, delivering industry-specific personalized responses while maintaining control.
Enhancing Customer Experience Through Personalization
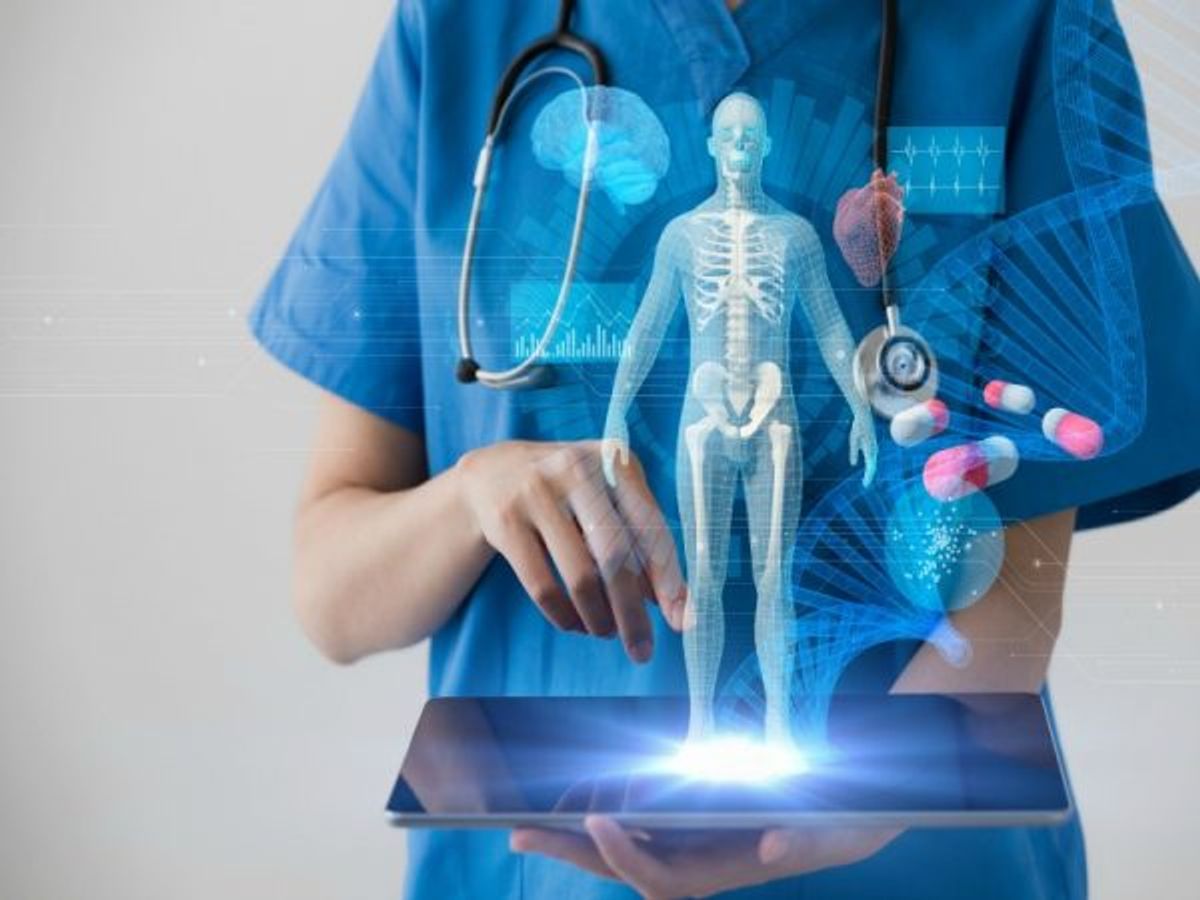
Crafting Realistic Customer Personas
Machine learning is pivotal in understanding customer behaviors and preferences at a granular level. By analyzing large datasets, AI systems can identify patterns and trends that human analysts might overlook. This capability enables businesses to create detailed and realistic customer personas, which are essential for personalizing customer interactions and refining marketing strategies.
- Collecting customer data from various touchpoints
- Analyzing the data to identify common characteristics
- Grouping customers into personas based on these characteristics
The creation of customer personas is not just about aggregating data; it's about transforming that data into actionable insights that drive engagement and loyalty.
These personas allow for more targeted and effective communication, as they reflect the diverse needs and preferences of a business's customer base. By leveraging AI to craft these personas, companies can ensure that their engagement strategies are not only data-driven but also highly relevant to each individual customer.
Predictive Analytics for Proactive Engagement
Predictive analytics is revolutionizing the way businesses interact with their customers. By analyzing data and identifying patterns, AI can forecast potential customer behaviors and enable companies to act before an issue arises. This proactive approach can transform customer experiences from reactive to anticipatory, ensuring that each interaction is timely and relevant.
Predictive models can determine the next-best action for a customer, whether it's a personalized offer or a timely piece of information, fostering a seamless and low-effort experience.
The use of predictive analytics allows for a dynamic customer engagement strategy that is not only personalized but also contextual. Here's how organizations are shifting:
- From reactive interaction to proactive engagement.
- From high-effort to low-effort experiences.
- From out-of-context to contextual actions.
For instance, a simple trigger for proactive engagement could be a contract renewal date, while more advanced triggers involve machine learning algorithms predicting customer needs based on behavior. This strategic shift is essential for businesses aiming to reduce churn and enhance customer loyalty.
Sentiment Analysis for Emotional Insight
Sentiment analysis stands at the forefront of understanding customer emotions, providing businesses with the ability to gauge reactions to their products and services. By analyzing customer feedback across various platforms, companies can quickly identify areas that trigger negative responses and reinforce those that generate positive ones. This emotional insight is crucial for tailoring interactions and ensuring customer satisfaction.
The process involves parsing through vast amounts of data, from social media comments to customer support interactions, to detect patterns and sentiments. The goal is to transform qualitative feedback into actionable insights. For instance, a surge in negative sentiment around a product feature can prompt immediate improvements or customer outreach.
The integration of sentiment analysis into customer response systems allows for a more nuanced understanding of the customer's voice, leading to more empathetic and effective communication.
The benefits of sentiment analysis are not just reactive but also proactive. By anticipating customer needs and emotions, businesses can craft personalized experiences that resonate on a deeper level, fostering loyalty and reducing churn.
Optimizing Interaction with Generative AI
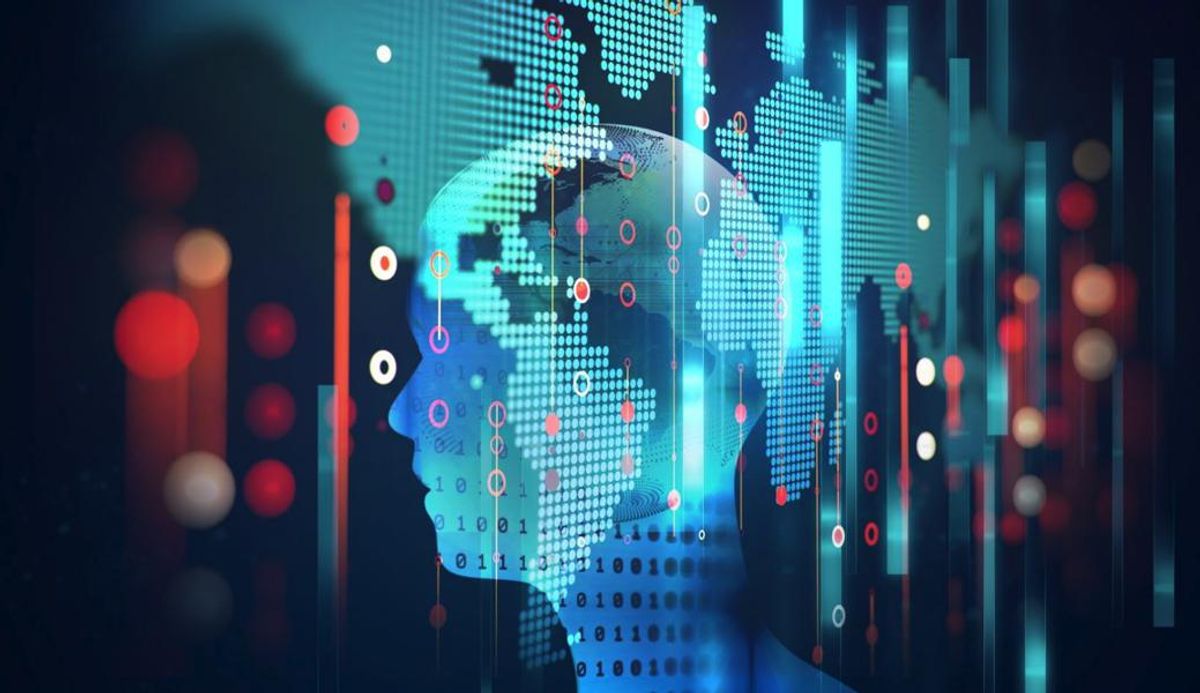
Knowledge and Personalization with Customer Data
The integration of customer data is pivotal in the realm of Generative AI, enabling systems to deliver tailored responses and guidance that resonate with individual preferences and behaviors. By leveraging insights from customer interactions, businesses can create a more personalized and engaging experience.
- Data Collection: Aggregating data from various touchpoints.
- Data Analysis: Utilizing machine learning to uncover patterns.
- Data Application: Applying insights to personalize interactions.
The convergence of customer knowledge and AI personalization is not just about enhancing the customer experience; it's about transforming it into a competitive advantage.
The use of Generative AI to personalize content and recommendations to each individual is a testament to the technology's potential in revolutionizing customer engagement. By crafting realistic customer personas and predicting behaviors, businesses can proactively address customer needs, thus reducing churn and increasing conversion rates.
Testing, Monitoring, and Observability of AI Models
Ensuring the reliability and effectiveness of Generative AI (GenAI) models is crucial for delivering personalized customer experiences. Regular testing and monitoring are essential to maintain the quality of AI-driven interactions. By analyzing real call data, businesses can observe the performance of their AI models and make necessary adjustments to improve accuracy and relevance.
The observability of AI models goes beyond mere functionality; it encompasses understanding the 'why' behind AI decisions, which is vital for trust and transparency.
To achieve this, a structured approach is necessary:
- Testing: Validate the AI model's responses against a diverse set of scenarios to ensure robustness.
- Monitoring: Continuously track the model's performance to detect any deviations or decline in quality.
- Observability: Implement tools that provide insights into the model's decision-making process, enabling quick identification and resolution of issues.
This iterative process helps in fine-tuning the AI models, ensuring that they remain aligned with organizational goals and customer expectations. As AI becomes more integrated into customer service platforms, the ability to adapt and evolve these models with changing business needs becomes a competitive advantage.
Prompt Library and Management for Efficient Development
The development of Generative AI (GenAI) applications is greatly expedited by having access to a comprehensive prompt library. This resource allows developers to quickly select and customize prompts that align with specific customer interactions, reducing the need for extensive prompt engineering expertise.
Effective management of this library is crucial, as it serves as a central hub for building, deploying, and testing GenAI-powered applications. By leveraging a centralized prompt management system, organizations can ensure that their AI models are not only accurate but also consistently updated to reflect the latest customer data and business insights.
The prompt library is a dynamic asset that requires regular updates and refinements to maintain its relevance and effectiveness in delivering personalized customer experiences.
Following best practices in prompt writing and management is essential for trustworthy GenAI applications. Experimentation and iterative development are key to refining AI outputs and ensuring they meet the high standards of precision and control demanded by today's businesses.
Strategies for Reducing Churn and Boosting Conversions
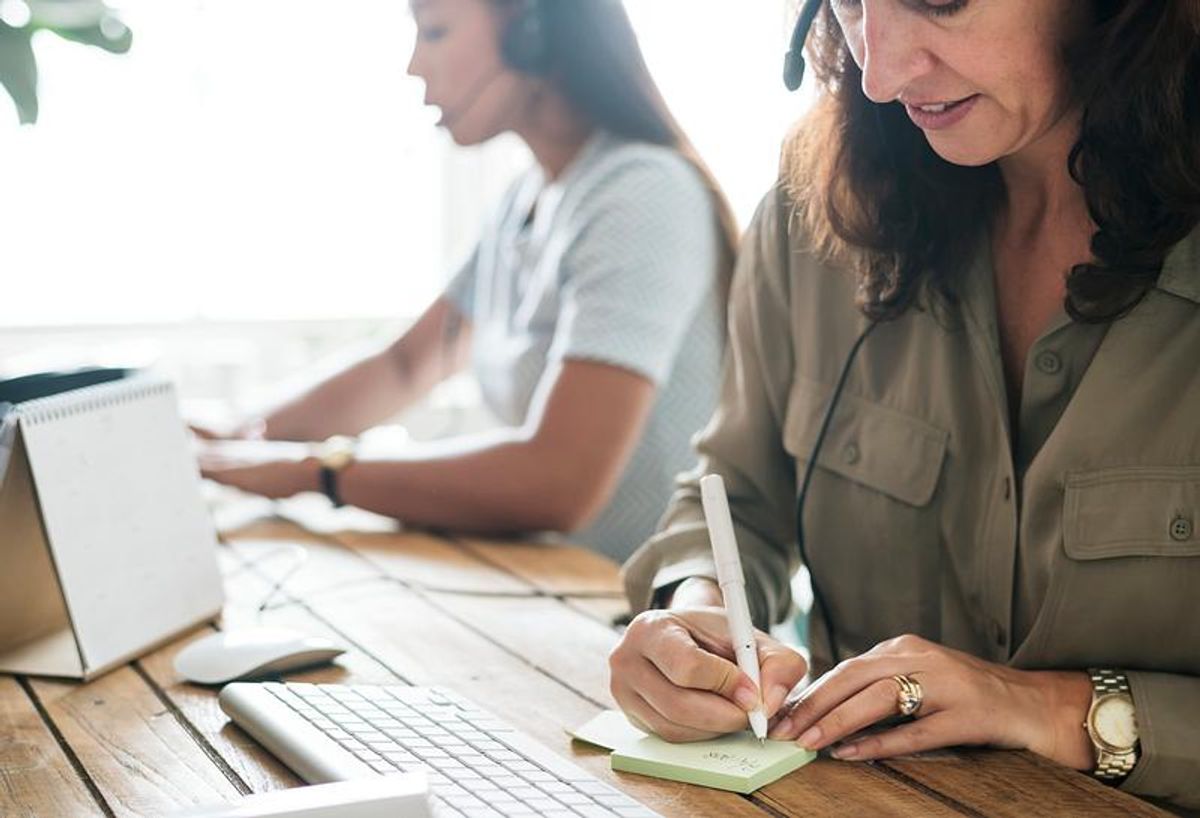
AI-Assisted Self-Service and Customer Experience
In the realm of customer service, AI-assisted self-service is a game-changer, offering a dual benefit of enhancing customer experience while reducing operational costs. By leveraging AI, businesses can provide personalized and instant support to customers, often without the need for human intervention. This not only improves the customer experience but also allows service teams to focus on more complex issues.
- AI-driven chatbots and virtual assistants can handle a multitude of customer queries in real-time.
- Predictive analytics enable proactive support, anticipating customer needs before they arise.
- Self-service portals powered by AI can guide customers through troubleshooting steps, reducing the need for direct contact.
The integration of AI into self-service solutions is not just about efficiency; it's about crafting a more responsive and tailored customer journey. By analyzing past interactions and preferences, AI can deliver a highly personalized service experience that resonates with the individual customer's needs.
The impact of AI on customer experience is evident in the numbers. For instance, a generative AI-enabled solution at JetBlue automated a significant portion of its chat channel, leading to substantial time savings and customer satisfaction. Similarly, Octopus Energy's use of AI for drafting email responses has resulted in higher customer contentment, showcasing the tangible benefits of AI in customer engagement.
Unlocking the Voice of the Customer
In the realm of customer experience, unlocking the voice of the customer is pivotal for businesses aiming to foster loyalty and drive growth. By leveraging machine learning algorithms, companies can now interpret vast amounts of customer feedback, extracting valuable insights that inform product development, marketing strategies, and customer service improvements.
The integration of AI into customer feedback analysis allows for a more nuanced understanding of customer needs and expectations.
The following points illustrate the transformative impact of AI on customer voice analysis:
- Sentiment analysis to gauge customer emotions and satisfaction levels.
- Intent recognition to anticipate customer needs and tailor responses.
- Trend identification to stay ahead of market shifts and customer preferences.
By systematically analyzing customer interactions, businesses can create a feedback loop that not only responds to but also anticipates customer needs, leading to enhanced satisfaction and reduced churn.
Omnichannel Integration and Revenue Growth
In today's competitive market, omnichannel integration is a cornerstone for achieving revenue growth. By leveraging multiple channels to interact with customers, businesses can create a seamless experience that fosters stronger relationships and encourages loyalty.
The key to successful omnichannel integration lies in the synchronization of data and communication across all platforms. This ensures that customers receive a consistent brand message and quality of service, regardless of the channel they choose to use.
- Enhanced Customer Experience
- Improved Brand Consistency
- Optimized Data-Driven Decision Making
- Increased Customer Engagement
By focusing on these core areas, companies can unlock significant growth and maintain a competitive edge in their respective industries.
Furthermore, the use of predictive analytics and AI-driven tools can lead to more informed and timely decisions, ultimately driving sales and reducing churn. The table below illustrates the impact of omnichannel strategies on key performance indicators (KPIs):
Embracing omnichannel strategies is not just about technology; it's about creating a culture that values customer engagement and understands the importance of a connected customer journey.
The Future of Customer Engagement

Hyper-Personalization in Customer Interactions
Hyper-personalization takes customer interaction to a new level, leveraging AI to tailor experiences uniquely for each individual. By analyzing vast datasets, businesses can now predict behaviors, enhance service quality, and create content that resonates on a personal level. This approach not only reduces churn but also significantly increases conversion rates.
- Out-of-context to contextual actions ensure customers receive relevant and trusted information when they need it.
- The shift from generic to personalized content heightens customer engagement.
- Transitioning from disconnected to connected journeys provides a seamless experience across channels.
- Evolving single interactions into continuous conversations fosters a deeper relationship throughout the customer lifecycle.
Hyper-personalization represents a paradigm shift in marketing, driven by the capabilities of AI and large language models. It's about understanding the individual customer on a deeper level and crafting experiences that are not just reactive but predictive and emotionally insightful.
Chatbots and Virtual Assistants in Retail and E-commerce
In the dynamic landscape of retail and e-commerce, chatbots and virtual assistants are revolutionizing the way businesses interact with customers. These AI-powered tools offer round-the-clock, multilingual support, enhancing customer service and loyalty. By analyzing customer data, chatbots deliver personalized responses that are contextually relevant and intent-driven, ensuring a seamless shopping experience.
Conversational AI, utilizing natural language processing (NLP), enables chatbots to conduct human-like conversations. They can perform complex tasks and, when necessary, seamlessly transfer queries to human agents. This blend of automation and personalization leads to improved service quality and increased operational efficiency.
The integration of AI into customer interactions is not just about efficiency; it's about creating a connection and understanding the customer on a deeper level.
According to Juniper Research, the use of AI-powered language models in conversational commerce is expected to drive retail spending through these channels to $43 billion by 2028. This represents a staggering growth, highlighting the critical role of AI in shaping future customer engagement strategies.
Continuous Conversations and Connected Customer Journeys
The evolution from single interactions to continuous conversations is a transformative shift in customer engagement. By fostering ongoing engagement through a series of communications, businesses can maintain a dynamic connection with their customers throughout the entire lifecycle. This approach not only nurtures loyalty but also paves the way for a more personalized experience.
Moving from out-of-context to contextual actions, customers now receive information and actions that are relevant, trusted, and timely. The transition from generic to personalized content significantly increases a customer's willingness to engage, as they feel understood and valued.
The seamless orchestration of customer journeys across multiple channels ensures that the conversation never has to start over. Customers appreciate the connectedness of their experiences, which in turn reduces churn and boosts conversions.
The integration of conversational AI into customer engagement strategies is not just about automating interactions; it's about creating a comprehensive conversational strategy that leverages customer insights across multiple channels. This is why automated conversations will be key to the customer experience, as they represent the organization's commitment to proactive engagement and low-effort experiences.
Responsible AI and Customization
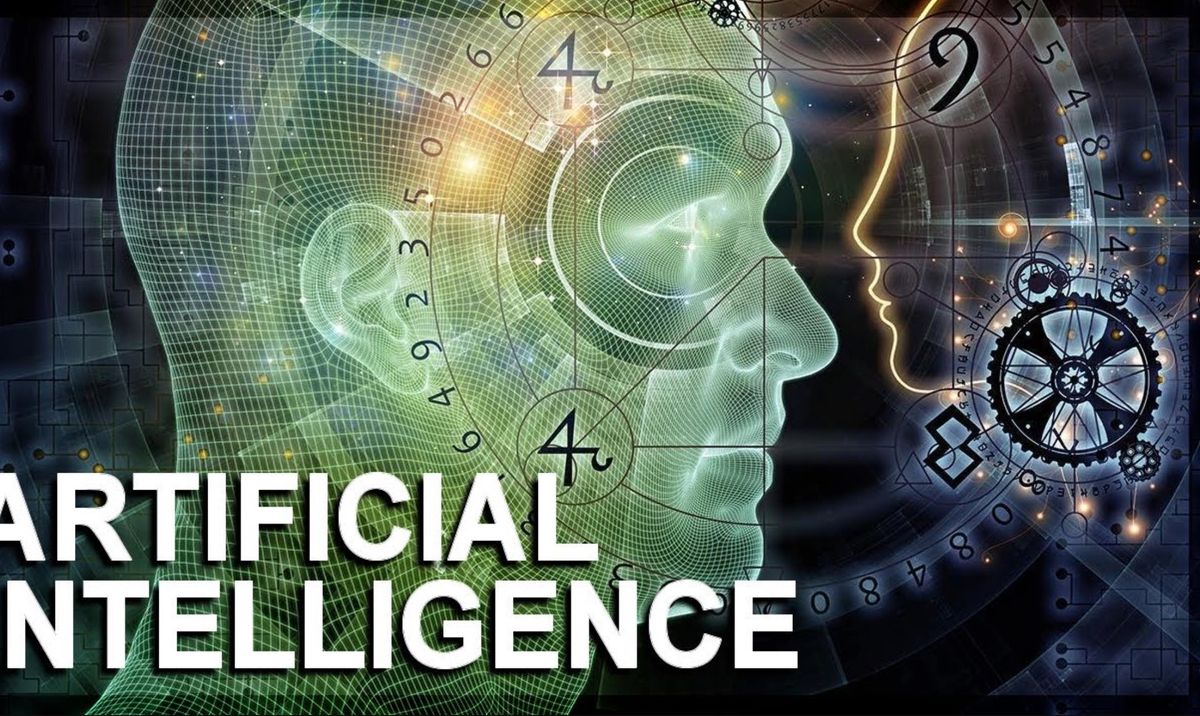
Ensuring Precision and Control in AI Outputs
In the realm of Responsible AI, precision and control are paramount. It requires employing a nuanced understanding of the customer's profile and past interactions, while adhering to clearly defined mission statements and rules. By providing precise control and monitoring of GenAI outputs, businesses can ensure that the customer experience is not only personalized but also consistent and reliable.
To achieve this, organizations must tailor their AI models and prompts to align with their unique needs. This involves customizing prompts, fine-tuning models, and adapting to changing business needs to maintain close alignment with organizational goals. The table below outlines key aspects of precision and control in AI outputs:
The goal is to deliver personalized, relevant interactions that resonate with each individual customer, thereby enhancing the overall customer experience.
With the right approach, businesses can unlock the potential of GenAI for customer experience (CX), generating human-like responses and insights that are not only impressive but also deeply aligned with the company's mission and customer expectations.
Adapting AI to Evolving Business Needs
As the landscape of customer engagement evolves, businesses must adapt their AI strategies to stay ahead. AI's ability to personalize experiences and predict behaviors is not just about saving time; it's about enhancing service quality and fostering customer loyalty. With AI, companies can leverage interaction data to tailor content, making every customer feel unique and valued.
- AI-driven predictive analytics can identify customers at risk of churn, prompting proactive engagement.
- Sentiment analysis tools powered by AI provide emotional insights, enabling businesses to quickly address issues.
- Generative AI helps in crafting effective communication, as seen with companies like Octopus Energy and JetBlue.
By embedding AI, we can improve the experience for consumers and brands across the customer journey, leading to higher ROI and cost savings.
Adapting AI to business needs means continuously testing and refining AI models to ensure they align with current customer expectations and market trends. It's about precision and control in AI outputs, ensuring that the technology serves the business strategy and not the other way around.
Industry-Specific Use Cases for Personalized Responses
The application of AI for personalized customer responses is as diverse as the industries it serves. In healthcare, AI leverages patient data to provide personalized treatment plans, considering genetic information and lifestyle factors. This not only enhances patient care but also streamlines the healthcare process.
In the realm of human resources, AI transforms recruitment by enabling personalized candidate communication. It ensures that potential employees receive tailored interactions, improving the recruitment experience and employer branding.
Retail and e-commerce have seen a revolution with AI chatbots offering round-the-clock, multilingual support. These chatbots are capable of understanding customer context and intent, providing a highly personalized shopping experience.
The key to successful AI implementation lies in its ability to adapt to the unique demands of each industry, ensuring that every customer interaction is as relevant and individualized as possible.
For businesses, the creation of realistic customer personas using AI analysis of large datasets is invaluable. It allows for a deeper understanding of customer needs and the ability to refine marketing strategies effectively.
Conclusion
In summary, machine learning is not just a tool for efficiency; it is a transformative force in customer engagement. By harnessing the power of AI, businesses can craft personalized customer responses that resonate on an individual level. The ability to analyze vast datasets and extract meaningful insights allows for the creation of realistic customer personas and the delivery of content that is not only relevant but also timely and context-aware. From reducing churn through predictive analytics to enhancing the overall customer experience with seamless, omnichannel interactions, the role of machine learning in customer service is proving to be invaluable. As we have seen, the integration of AI into customer response systems leads to more than just operational efficiency—it fosters deeper customer relationships and drives business growth. The future of customer engagement is undeniably intertwined with the continued advancement of AI, and businesses that embrace this technology will be well-positioned to thrive in the competitive landscape.
Frequently Asked Questions
How does machine learning enhance personalized customer experiences?
Machine learning enhances personalized customer experiences by leveraging large datasets to craft realistic customer personas, predict behaviors, and tailor content and recommendations to individual needs. This leads to higher service quality and improved engagement.
What role does sentiment analysis play in customer engagement?
Sentiment analysis helps businesses understand customer emotions toward their brand by analyzing feedback across various platforms. This enables companies to quickly address issues, reinforce positive aspects, and adjust their strategies for better engagement.
How can AI reduce churn and increase conversion rates?
AI can identify customers at risk of churn through predictive analytics, enabling businesses to take proactive measures such as offering tailored deals to retain them. Additionally, AI-powered personalized interactions can improve service quality and drive conversions.
What are the benefits of integrating conversational AI in customer service?
Conversational AI can automate and personalize customer interactions, leading to enhanced service quality, increased operational efficiency, and round-the-clock, multilingual support that drives customer loyalty and lead generation.
How does GenAI Studio contribute to knowledge and personalization with customer data?
GenAI Studio integrates customer data to provide tailored responses and guidance. It also offers testing, monitoring, and observability of AI models, along with a prompt library and management tools to expedite AI-driven development.
What are the key factors for responsible AI in customization?
Responsible AI in customization involves providing precise control and monitoring of AI outputs, delivering personalized interactions with tailored prompts, and adapting to changing business needs to maintain close alignment with organizational goals.