Artificial intelligence (AI) has become a pivotal player in the energy sector, not only by optimizing energy systems but also by transforming customer relationships. This article delves into the multifaceted role of AI in sustainable energy, examining how it enhances grid efficiency, integrates renewable sources, and improves customer engagement. By leveraging AI and big data, energy companies are redefining their operations and customer service, paving the way for a more efficient, sustainable, and customer-centric energy future.
Key Takeaways
- AI enables more accurate forecasting of energy demand and facilitates efficient grid operations, contributing to a sustainable energy landscape.
- Through machine learning and predictive analytics, AI aids in the integration of renewable energy sources and optimizes load balancing.
- AI-driven insights help in developing low-emission infrastructure and enhance carbon capture, addressing climate change challenges.
- Enhanced customer relations through AI include personalized energy services and data-driven engagement, fostering trust and satisfaction.
- Policy and regulatory frameworks need to evolve to support AI innovation in energy while ensuring data protection and ethical standards.
Optimizing Energy Systems with AI

Forecasting Demand and Enhancing Grid Efficiency
The integration of AI in energy systems is revolutionizing the way we manage and distribute power. Advanced machine learning algorithms and neural networks are at the forefront of this transformation, enabling more accurate forecasting of demand surges and enhancing grid efficiency. This not only ensures a more reliable energy supply but also paves the way for a smarter, more sustainable infrastructure.
- AI-driven analytics provide deep insights into consumption patterns.
- Machine learning optimizes the distribution networks, identifying inefficiencies.
- Neural networks enhance the accuracy of demand forecasting.
The sustainable urban energy management model of Masdar City serves as a prime example of how AI can be utilized to optimize energy systems. Here, AI technologies are employed to minimize energy waste and improve load balancing, demonstrating the practical benefits of such integration.
The transformative impact of AI extends beyond mere efficiency; it is reshaping the entire energy landscape. By leveraging big data and AI-driven decision-making, the energy sector is moving towards a future where energy management is not only sustainable but also more attuned to the dynamic needs of the grid and its consumers.
Integrating Renewable Energy Sources
The integration of renewable energy sources into power systems is pivotal for creating a sustainable energy future. Storage solutions play a crucial role in this integration, enabling the balancing of supply and demand and ensuring the reliability of the grid. As renewable sources like solar and wind are intermittent, energy storage systems are essential for maintaining a steady supply of power.
The seamless integration of renewables, storage solutions, and smart devices across the energy grid is a cornerstone of a resilient and efficient energy system.
To achieve this integration, a multi-faceted approach is necessary, involving:
- The development and implementation of interoperability standards.
- Collaborative efforts between governments, industry, and standardization bodies.
- Ethical considerations in the use of AI and data to manage these integrated systems.
These efforts collectively contribute to an energy grid that is not only more sustainable but also more responsive to the needs of consumers.
AI-Driven Decision Making for Load Balancing
The deployment of AI in smart grid management enables real-time monitoring and decision-making, optimizing the balance between energy supply and demand. This not only enhances grid efficiency but also paves the way for a more sustainable energy sector.
The integration of AI and big data analytics has revolutionized the way we approach energy management. It allows for the minimization of energy waste and the improvement of load balancing across energy grids.
By utilizing advanced machine learning algorithms and neural networks, AI-driven systems can accurately forecast demand surges, ensuring that energy distribution is optimized. This leads to a more resilient grid that can adapt to changing consumption patterns and integrate renewable energy sources more effectively.
The following points highlight the benefits of AI in load balancing:
- Reduction in energy waste
- Enhanced ability to integrate renewable sources
- Improved reliability and efficiency of the grid
- Support for sustainable urban energy management models, like that of Masdar City
AI in Energy Efficiency and Carbon Emission Reduction

Smart Grid Design and Operation
The evolution of the electric power grid has reached a pivotal moment with the integration of AI-enhanced smart grid technologies. These advancements promise to revolutionize the energy sector by providing enhanced efficiency and reliability through automated control systems, high-power converters, and modern communications infrastructure. The smart grid represents a significant leap from the traditional grid, which has remained largely unchanged for a century, to a dynamic and responsive network capable of meeting the demands of the 21st century.
Key components of this transformation include the integration of various elements of the energy grid, from renewable energy sources and storage systems to consumer devices like smart meters and home energy management systems. The standardization of technical standards is crucial for ensuring that these systems communicate and operate seamlessly, guaranteeing compatibility and interoperability across different technologies and platforms.
The smart grid is not just an upgrade of the existing infrastructure; it is a complete re-envisioning of energy distribution and management, leveraging the power of AI to optimize demand, energy, and network availability.
The table below outlines the core aspects of smart grid design and operation:
Low-Emission Infrastructure Development
The development of low-emission infrastructure is pivotal in the transition towards a sustainable energy future. Public-Private Partnerships (PPPs) have emerged as a key mechanism to drive this development, combining public goals with private sector efficiency and innovation. These partnerships can mobilize significant private investment and expertise, reducing the financial and operational burden on public entities while accelerating the rollout of innovative energy solutions.
By fostering successful PPPs, we can enhance energy efficiency, expand renewable energy capacity, and improve grid reliability, all of which are essential for achieving long-term sustainability and energy security goals.
The role of Energy Service Companies (ESCOs) is also crucial. ESCOs assume the responsibility of financing and implementing energy-saving measures, such as lighting upgrades and HVAC system optimizations. The investment made by ESCOs is recouped through the energy cost savings over a contractually defined period, aligning the financial interests towards reducing energy consumption.
Here are some key aspects of PPPs in low-emission infrastructure development:
- Enhancing energy efficiency
- Expanding renewable energy capacity
- Improving grid reliability
- Transparent procurement and competitive bidding
- Performance-based contracts
Machine Learning in Carbon Capture and Storage
Machine learning (ML) is revolutionizing the field of carbon capture and storage (CCS), offering innovative ways to tackle climate change. By predicting optimal storage locations and improving capture processes, ML algorithms are instrumental in enhancing the efficiency of CCS operations. These intelligent systems analyze vast datasets to identify patterns and optimize the entire CCS value chain.
- ML models simulate various scenarios to find the most efficient strategies for carbon capture.
- Continuous data analysis helps in proactive problem-solving and rapid operational adjustments.
- Predictive analytics are used to assess risks and ensure safety in CCS projects.
While AI and ML present significant opportunities for carbon emission reduction, it is crucial to acknowledge the energy-intensive nature of these technologies. The balance between their benefits and carbon footprint must be carefully managed to ensure a net positive impact on the environment.
The Impact of AI on Energy Consumption Patterns
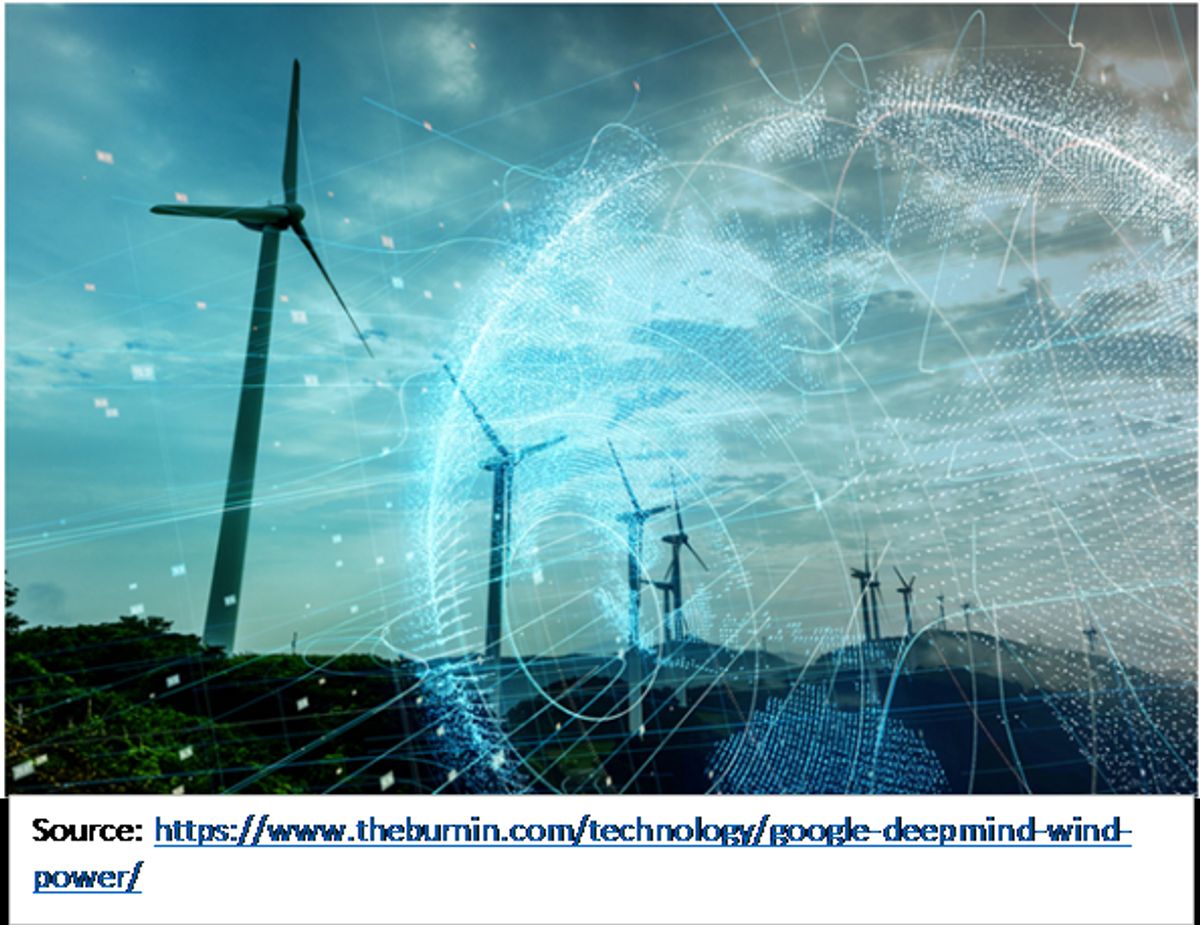
Predictive Analytics for Energy Management
The integration of predictive analytics into energy management systems marks a significant leap forward in optimizing energy consumption and grid efficiency. By leveraging machine learning algorithms and extensive data analytics, energy companies can accurately forecast demand, reduce waste, and enhance grid operations. This proactive approach allows for more precise adjustments to energy supply, ensuring that energy generation aligns closely with consumption patterns.
- Demand Forecasting: Predictive models analyze historical and real-time data to anticipate energy usage trends.
- Waste Reduction: Identifying inefficiencies helps in minimizing energy loss and reducing operational costs.
- Grid Optimization: AI enables dynamic adjustments to energy distribution, improving overall grid reliability.
The sustainable urban energy management model of Masdar City exemplifies the practical benefits of predictive analytics in action, showcasing how AI can drive smarter, more efficient energy systems.
The challenges of data privacy and ethical considerations remain paramount as we harness the power of AI in energy management. It is crucial to balance the technological advancements with responsible data stewardship to maintain consumer trust and comply with regulatory standards.
The Rebound Effect of Technological Progress
The rebound effect represents a paradox in the sustainable energy sector. As technological advancements make energy systems more efficient, the lower costs can lead to increased energy consumption, offsetting some of the anticipated energy savings. This effect challenges the notion that efficiency alone can lead to significant reductions in energy use.
The concept of the rebound effect is important in understanding the limits to how much technological efficiency improvements can reduce energy and resource consumption.
However, the rebound effect is only part of the narrative. It is crucial to consider the broader implications of technological progress, including how it can spur innovation and drive the adoption of cleaner energy solutions. For instance, AI can optimize the integration of renewable energy sources, leading to a more sustainable energy mix. Below is a list of ways AI can mitigate the rebound effect:
- Monitoring energy consumption patterns to identify and discourage wasteful practices.
- Automating the operation of energy systems to ensure optimal efficiency.
- Incentivizing energy-saving behaviors through dynamic pricing and rewards programs.
- Educating consumers about the environmental impact of their energy choices.
Balancing Supply and Demand with AI
The integration of AI into energy management systems is revolutionizing the way supply and demand are balanced. AI's predictive analytics capabilities enable energy providers to anticipate demand spikes and adjust supply accordingly. This not only ensures a stable energy grid but also contributes to cost savings and reduced waste.
AI's role in balancing supply and demand extends beyond mere prediction. It actively facilitates the dynamic adjustment of energy flows, optimizing the use of resources and enhancing the overall efficiency of the energy sector.
AI-driven systems can automate the procurement process, ensuring that energy resources are ordered and supplied just in time, minimizing stockouts and excess inventory. This level of automation streamlines contract management and invoicing processes, leading to more favorable supplier agreements and improved cash flow management.
- Monitor and adjust energy distribution in real-time
- Predict and manage demand surges
- Optimize procurement and inventory
- Streamline contract management
- Enhance supplier relationships
AI-Enhanced Customer Relations in the Energy Sector
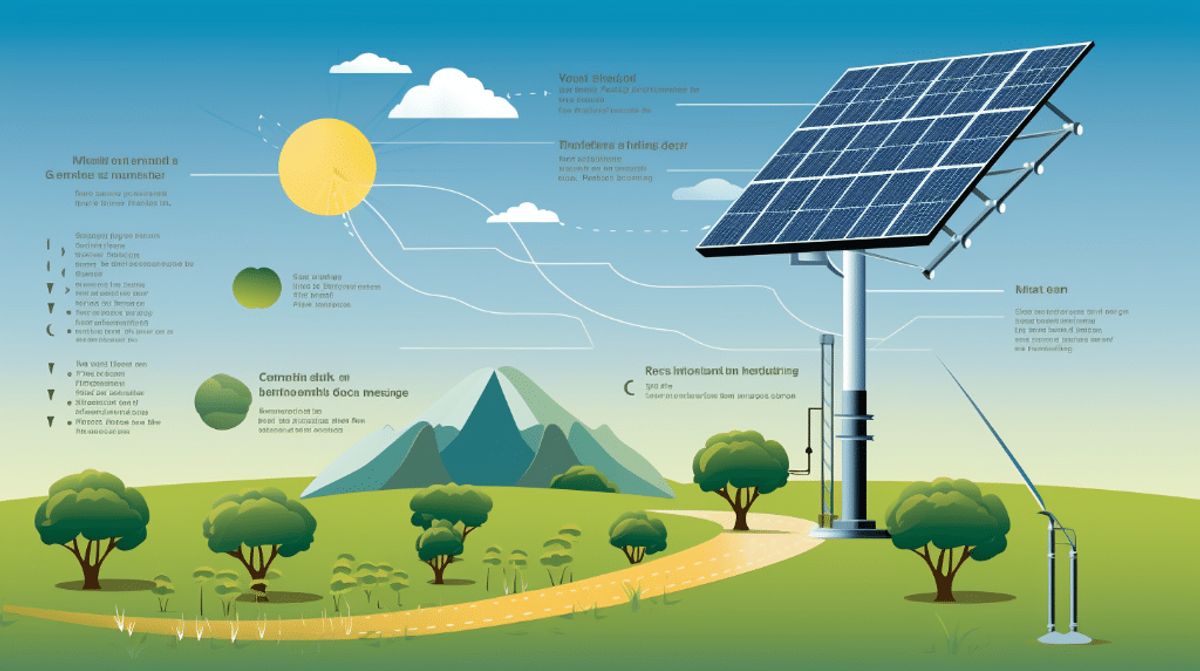
Personalized Energy Services
In the realm of sustainable energy, AI is revolutionizing the way customers interact with service providers. Personalized energy services, powered by AI, are not just a futuristic concept but a present reality. These services leverage customer data to tailor energy solutions that align with individual consumption patterns, preferences, and even budget constraints.
- Forecasting individual energy needs allows for more accurate billing and customized energy-saving tips.
- Smart home integrations enable automated adjustments to heating, cooling, and lighting, optimizing energy use without sacrificing comfort.
- Dynamic pricing models offer cost savings by encouraging energy use during off-peak hours.
By harnessing the power of AI, energy companies can offer a suite of personalized services that not only enhance customer satisfaction but also contribute to overall energy efficiency.
The adoption of AI in providing personalized energy services is a testament to the sector's commitment to innovation. It reflects a shift towards a more customer-centric approach, where the benefits of AI in the energy sector—such as strengthened efficiency and reduced operational costs—are passed directly to consumers.
Improving Customer Engagement through Data Insights
The integration of AI in the energy sector has enabled companies to leverage vast datasets to predict customer behaviors and deliver personalized experiences. By analyzing consumption patterns, energy providers can tailor their services to meet individual needs, fostering a more engaged customer base.
- Understanding consumption trends
- Identifying opportunities for energy savings
- Offering customized energy solutions
AI-driven insights not only enhance customer satisfaction but also encourage sustainable energy practices by promoting efficiency.
The use of AI in customer engagement is not just about data analysis; it's about transforming that data into actionable strategies that resonate with consumers. Energy companies that harness these insights can build stronger, more loyal relationships with their customers.
Building Trust with Ethical AI Practices
In the pursuit of ethical AI practices, energy companies are increasingly focusing on the delicate balance between automation and the human touch. A responsible AI strategy in customer service not only streamlines operations but also ensures that empathy and understanding remain at the forefront of customer interactions. By prioritizing data privacy and transparency, companies build a foundation of trust with their consumers.
The integration of AI into customer relations must be handled with care to avoid depersonalization. Ensuring human oversight in AI systems is crucial for maintaining a connection with customers that feels genuine and considerate.
To further cement trust, energy companies can adopt the following measures:
- Monitor supply chains for ethical compliance, ensuring that labor rights and environmental impacts are respected.
- Automate due diligence processes to reduce the risk of overlooking ethical concerns.
- Analyze supplier behavior to predict and prevent potential ethical issues.
These steps, when implemented effectively, demonstrate a commitment to ethical procurement and sustainable practices, aligning with broader ESG goals. As AI continues to evolve, maintaining these ethical standards will be key to fostering long-term customer relationships.
Policy and Regulatory Considerations for AI in Energy
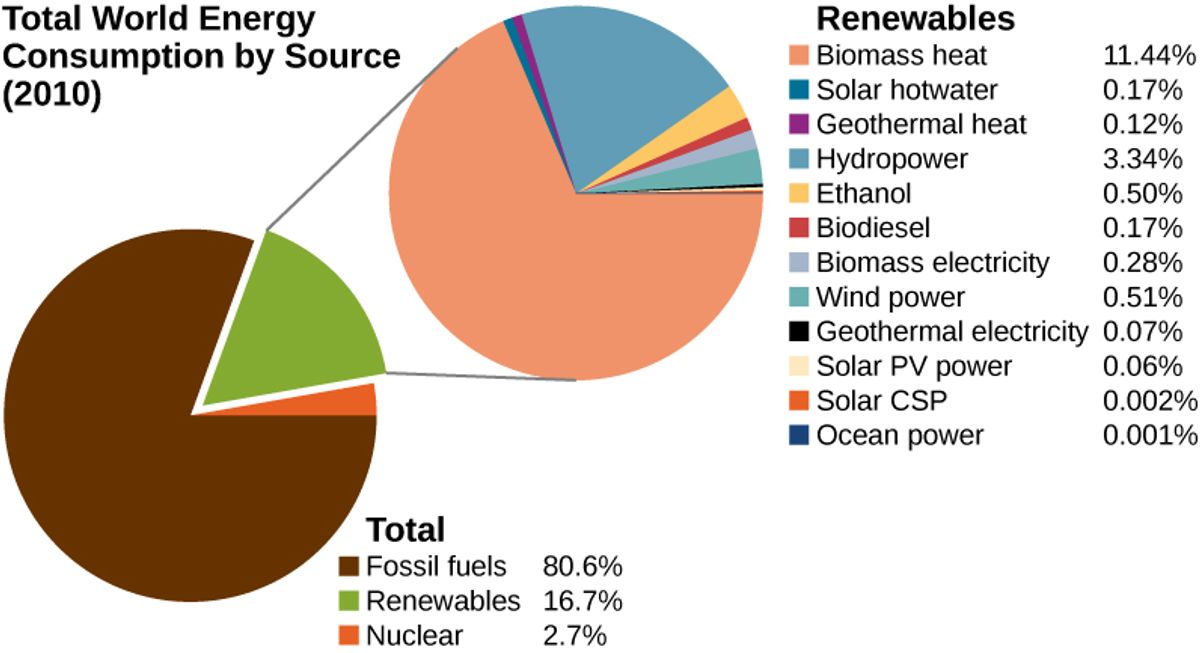
Creating Supportive Frameworks for Innovation
The development of supportive frameworks is a cornerstone for fostering innovation in the energy sector. Regulatory sandbox environments are instrumental in this regard, allowing for the testing of new technologies under real-world conditions with a degree of regulatory flexibility. This approach not only accelerates the adoption of innovative solutions but also provides critical insights into their performance and potential impacts.
The establishment of collaborative platforms is another key strategy. These platforms facilitate the sharing of information, resources, and best practices among stakeholders, creating an environment of transparency and mutual understanding that is conducive to innovation.
To further enhance innovation, it is crucial to set clear standards and guidelines. These provide a technical framework within which innovators can operate, ensuring interoperability and fostering consumer choice. The table below outlines the benefits of standardization in the energy sector:
By prioritizing these elements, we can create an ecosystem that not only supports current innovation but also paves the way for future advancements in sustainable energy.
Data Protection and Privacy in AI Applications
As the energy sector transitions to intelligent systems powered by AI, ensuring the privacy and security of data is not just a technical challenge but a fundamental aspect of maintaining public trust. The deployment of AI in energy management involves the collection and analysis of vast amounts of data, which can include sensitive personal information. Protecting this data against unauthorized access and misuse is paramount.
To address these concerns, a multi-layered approach to data privacy is essential:
- Data Protection Measures: Implementation of robust security protocols such as encryption and access controls.
- Regulatory Compliance: Adherence to data protection laws and industry standards.
- Transparency: Clear communication with stakeholders about how data is used and protected.
- Ethical AI Practices: Ensuring AI systems are designed with respect for privacy and human rights.
The integration of AI into the energy sector brings immense benefits, but it must be balanced with the responsibility to protect individual privacy and uphold ethical standards.
The development and enforcement of targeted data protection laws, along with the adoption of advanced encryption and anonymization techniques, are critical steps in this process. Stakeholders must collaborate to establish a robust framework that not only fosters innovation but also safeguards against the risks associated with data breaches and cyber-attacks.
Navigating the Transition to Intelligent Energy Systems
The shift towards intelligent energy systems is a complex process that requires careful navigation. Regulatory flexibility is essential in adapting to the rapid advancements in smart energy technologies. This flexibility ensures that regulations not only support innovation but also protect public interest and system integrity.
The integration of AI and big data analytics into energy systems demands a dynamic regulatory approach. Sandbox environments and collaborative platforms are instrumental in fostering innovation while ensuring a smooth transition.
Stakeholder engagement is another critical aspect of this transition. It is vital to:
- Establish collaborative platforms for stakeholder dialogue
- Build trust in smart energy systems
- Encourage the adoption of sustainable and efficient energy consumption practices
Ultimately, the successful transition to integrated, intelligent energy systems hinges on the synergy between technological innovation, regulatory frameworks, and stakeholder cooperation.
Conclusion
In conclusion, the integration of artificial intelligence (AI) into the energy sector stands as a pivotal development in the pursuit of sustainability and enhanced customer relations. AI's capacity to analyze vast datasets and make predictive decisions is revolutionizing energy management, from optimizing grid operations to facilitating the adoption of renewable energy sources. This paper has highlighted the transformative impact of AI on energy efficiency, carbon emission reduction, and the balance of supply and demand in power systems. However, it also acknowledges the complexities and potential rebound effects that AI may introduce. As we advance, it is crucial to foster collaboration among all stakeholders and to establish regulatory frameworks that support ethical and efficient AI integration. The journey towards a sustainable energy future is indeed multifaceted, and AI is an indispensable ally in navigating this terrain, promising a smarter, more responsive, and environmentally conscious energy landscape.
Frequently Asked Questions
How does AI contribute to optimizing energy systems?
AI contributes to optimizing energy systems by forecasting demand, improving grid efficiency, integrating renewable energy sources, and making AI-driven decisions for load balancing, thus minimizing energy waste and enhancing overall system performance.
What role does AI play in reducing carbon emissions in the energy sector?
AI plays a significant role in reducing carbon emissions by enabling smart grid design, facilitating the development of low-emission infrastructure, and improving machine learning applications in carbon capture and storage, which helps in identifying optimal storage locations and optimizing the capture process.
How can AI impact energy consumption patterns?
AI impacts energy consumption patterns through predictive analytics for energy management, which helps in anticipating and adjusting to energy needs. However, it can also lead to a rebound effect where increased efficiency may stimulate further energy use, potentially reducing the expected savings.
In what ways does AI enhance customer relations in the energy sector?
AI enhances customer relations by providing personalized energy services, improving customer engagement with data insights, and building trust through the implementation of ethical AI practices, which ensure transparency and privacy.
What are the policy and regulatory considerations for AI in the energy sector?
Policy and regulatory considerations include creating frameworks that support innovation, ensuring data protection and privacy in AI applications, and managing the transition to intelligent energy systems in a way that aligns with ethical standards and public expectations.
Can AI help in integrating renewable energy sources into the power grid?
Yes, AI can significantly assist in the integration of renewable energy sources into the power grid by predicting solar and wind patterns, optimizing inverter control for photovoltaic systems, and managing generation schedules, thus ensuring a stable and efficient energy supply.