The integration of Artificial Intelligence (AI) into customer service, particularly within the legal tech industry, presents a complex array of challenges. From defining the scope of AI implementation to ensuring seamless integration with existing systems, companies must navigate technical, operational, and financial hurdles to harness the transformative power of AI. This article delves into the multifaceted process of implementing AI in customer service, offering insights into the strategic process selection, team restructuring, and financial considerations that are crucial for success.
Key Takeaways
- Clarifying objectives and ensuring high-quality data are foundational steps in the AI implementation framework.
- Technical and operational challenges, such as interoperability and team structure, must be addressed to optimize AI integration.
- Strategic process selection is crucial, with a focus on automating high-impact processes and customizing AI for specific legal tech needs.
- Financial considerations involve a comprehensive approach to estimating costs and aligning AI investments with business value.
- Successful AI integration in legal tech requires methodical analysis, collaborative development, and a commitment to continuous improvement.
Defining the AI Implementation Framework
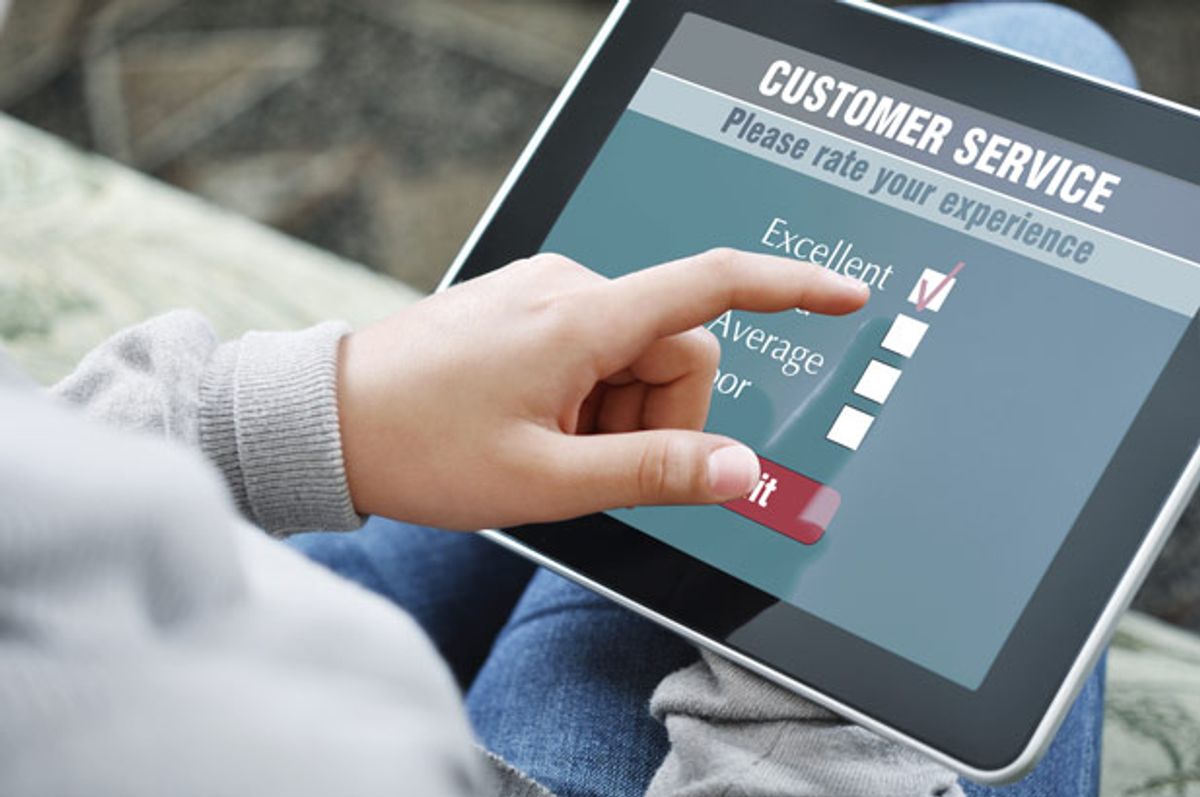
Clarifying Objectives and Goals
The foundation of successful AI implementation in customer service begins with clearly defining objectives and goals. This clarity is essential not only for aligning AI initiatives with organizational needs but also for setting realistic expectations for what AI can achieve. Ambiguity in objectives can lead to misaligned efforts and wasted resources.
Establishing a well-defined scope is critical to the success of AI projects. It guides the development process and ensures that the AI solutions developed are in sync with the strategic vision of the organization.
The initial considerations often include ambiguous goals, which after careful analysis and stakeholder involvement, transform into actionable and aligned objectives. Here is a comparison of the scope considerations before and after intervention:
By addressing these key areas, organizations can ensure that their AI initiatives are built on a solid foundation, with a clear direction and purpose.
Ensuring Data Quality and Availability
The foundation of any successful AI implementation in customer service is the assurance of high-quality and readily available data. Data quality issues such as incomplete, inaccurate, or biased datasets can severely undermine the training and performance of AI models. To combat these challenges, it is essential to implement robust data governance policies that address concerns related to data quality, privacy, and compliance.
A strategic approach to data management includes:
- Establishing clear criteria for data collection and processing
- Regularly auditing data for accuracy and completeness
- Implementing mechanisms for data cleansing and enrichment
Ensuring data integrity is not just a technical necessity but a strategic imperative that underpins the entire AI deployment process.
Collaboration with technology teams is crucial to determine the best strategies for data transfer and storage, whether it be cloud-based or on-premise solutions. This collaboration ensures that technical decisions are aligned with the broader business objectives and that the AI system is scalable and efficient.
Assessing Process Complexity
When implementing AI in customer service, assessing the complexity of processes is crucial. Highly intricate or dynamic processes may require sophisticated AI solutions, impacting both development and maintenance costs. Resource constraints, including limited budget and skilled personnel, also influence the scope of AI implementation. Balancing ambitions with available resources is a persistent challenge.
Designing an efficient and scalable integration architecture involves a comprehensive analysis of the customer's existing systems and software components. Collaborative sessions with key stakeholders are facilitated to understand the intricacies of the workflow, identify critical integration points, and assess specific requirements for efficiency and scalability.
Scalability concerns add to the complexity. Striking the right balance between scalable solutions and cost considerations is crucial. Ethical and regulatory considerations further shape the scope, requiring careful evaluation of legal and ethical implications.
The following table outlines the initial scope considerations versus the finalized scope after strategic interventions:
Balancing Resources and Ambitions
When venturing into AI implementation, organizations must carefully balance their ambitions with available resources. The allure of advanced AI capabilities often leads to overly ambitious projects that overlook the practical constraints of budget and skilled personnel. To avoid common pitfalls, it's essential to implement AI strategically, as suggested by Netguru's advice to overcome mistakes and leverage software agencies for successful integration.
Scalability concerns further add to the complexity. Striking the right balance between scalable solutions and cost considerations is crucial. Overly ambitious scalability goals may lead to higher upfront costs, while minimal consideration of ethical and regulatory aspects can result in compliance issues down the line.
Here is a comparison of initial scope considerations versus the finalized scope after involvement:
Optimizing AI maintenance costs is not just about reducing expenses but ensuring that the AI system can grow with the organization without necessitating frequent, costly overhauls.
Overcoming Technical and Operational Hurdles
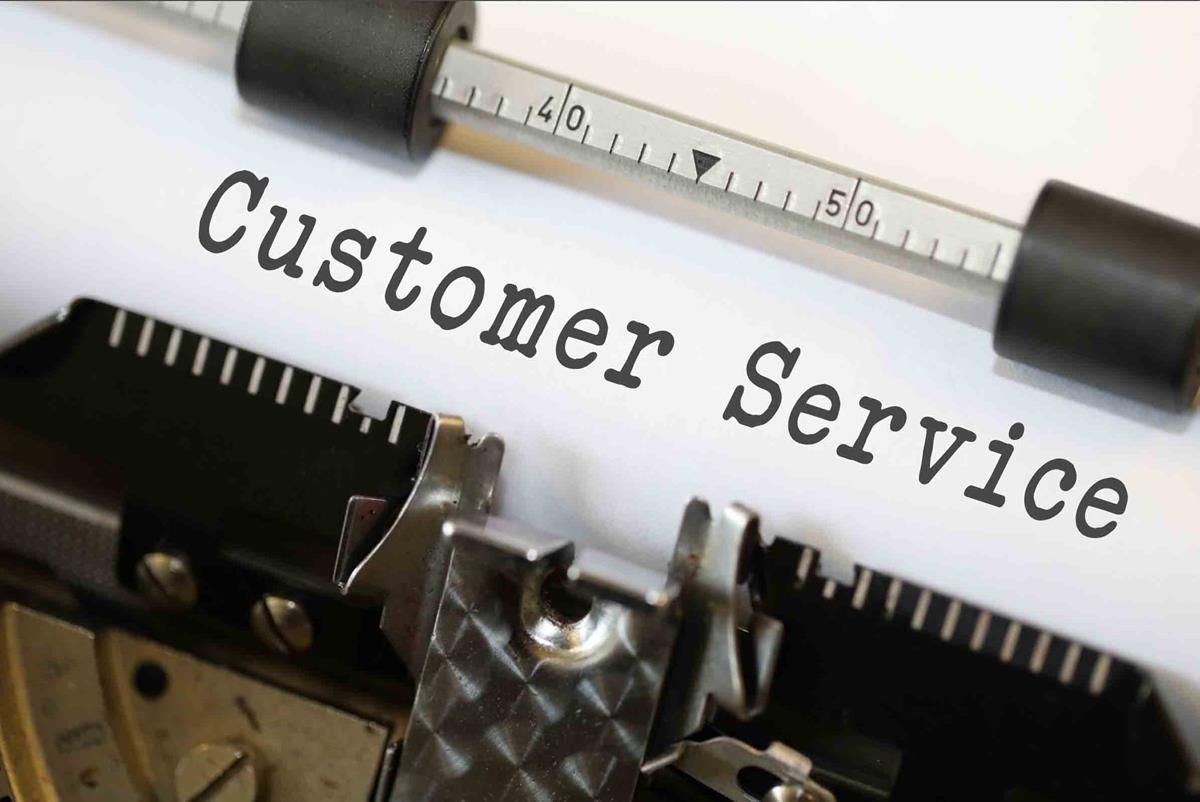
Addressing Interoperability with Existing Systems
Ensuring that AI systems work harmoniously with existing infrastructure is a pivotal step in AI implementation. Compatibility challenges, version management, and coordination among various systems can significantly complicate integration efforts. A well-designed integration architecture is essential for overcoming these hurdles.
- Begin with a comprehensive analysis of current systems and software components.
- Facilitate collaborative sessions with stakeholders to understand workflow intricacies and identify critical integration points.
- Engage with the technology team to discuss server locations and decide between cloud-based and on-premise solutions.
Fostering open communication and collaboration is crucial for aligning AI integration with the organization's broader technology strategy, ensuring performance, security, and cost-effectiveness.
Evaluating the integration capabilities of existing systems is necessary to determine the feasibility of seamless AI integration. Prioritizing opportunities for AI intervention should be based on potential impact, feasibility, and organizational goals alignment.
Optimizing Team Structure for AI Readiness
In the journey to AI readiness, the team structure is pivotal. A systematic approach is crucial, beginning with a skills assessment to identify gaps. Addressing these gaps can be achieved through targeted recruitment or comprehensive training programs.
- Skills Assessment
- Gap Identification
- Recruitment/Training
The transformation often requires integrating new roles, such as data scientists and AI specialists, to bridge the expertise gap. Moreover, the implementation of "Ops" functions can streamline processes and automate mundane tasks, freeing up the team to focus on high-value activities.
In the process of restructuring, it's essential to consider both the current and future needs of the AI initiatives to ensure a scalable and flexible team composition.
The table below illustrates the before and after scenario of a team's restructuring effort:
This approach not only prepares the team for current AI projects but also equips them to adapt to evolving AI landscapes.
Navigating Data Science Skill Gaps
The integration of AI into customer service is often hindered by a significant skills gap in data science. Companies face the challenge of either recruiting new talent with the necessary expertise or upskilling their current workforce. To address this, a targeted training strategy is essential.
- Initial Scope Considerations: Ambiguous objectives and goals, lacking clarity.
- Finalized Scope After Involvement: Clearly defined objectives aligned with organizational needs.
The transformation begins with identifying the gaps in AI expertise and then enhancing it through recruitment or training.
A structured approach to closing the skills gap involves several key steps:
- Initiate Transformation: Address limited AI expertise by identifying gaps.
- Encourage Collaboration: Foster improved cross-disciplinary collaboration.
- Define Roles and Responsibilities: Ensure clear roles to minimize confusion and foster accountability.
Ensuring Scalable AI Infrastructure
When integrating AI into customer service, scalability is a cornerstone for long-term success. Scalable AI infrastructure is essential to accommodate growth and evolving customer needs. It's not just about having the capacity to handle increased loads; it's also about maintaining performance, security, and cost-effectiveness at scale.
- Optimizing AI Maintenance Costs: It's crucial to understand the full spectrum of costs associated with AI, from initial setup to ongoing maintenance. A scalable AI infrastructure must be cost-effective, ensuring that the investment remains sustainable as the system grows.
- Collaborative Planning: Working closely with technology teams is key to determining the most effective server locations and deciding between cloud and on-premise solutions. This collaboration ensures that technical decisions support the broader business goals.
- Methodical Cost Estimation: A comprehensive approach to estimating AI maintenance costs involves understanding the specific needs of the system, such as computing power and storage requirements, to ensure scalability.
With all these requirements in place, scalable AI can help business operations move with more speed, security, accuracy, personalization, and even creativity.
Scalability considerations should be an integral part of the AI implementation framework from the outset. This includes assessing the initial scope and ensuring that objectives, data quality, and resource allocation are aligned with the potential for future expansion.
Strategic Process Selection for AI Enhancement

Identifying Automation Opportunities
To harness the full potential of AI in customer service, it's crucial to identify opportunities within your existing processes. Begin by gaining a comprehensive understanding of business operations, pinpointing tasks that are repetitive, rule-based, and prone to human error. An audit of these processes, coupled with a detailed data analysis, lays the groundwork for AI implementation.
Once the groundwork is laid, evaluate the integration capabilities of your systems. This step ensures that any AI solution can seamlessly interface with existing software or databases. With a clear picture of the operational landscape, you can then identify and prioritize opportunities for AI intervention. These should be aligned with organizational goals and assessed for their potential impact and feasibility.
The identification and prioritization of AI automation opportunities is a strategic step that sets the stage for transformative customer service enhancements.
Challenges such as the complexity of processes and integration with existing systems must be navigated carefully. By methodically assessing these factors, businesses can make informed decisions on which processes are most suitable for automation, thereby optimizing the impact of AI in customer service.
Evaluating Process Complexity for AI Suitability
When considering the integration of AI into customer service, the complexity of processes cannot be overlooked. Highly intricate or dynamic processes may require sophisticated AI solutions, impacting both development and maintenance costs. It's essential to balance ambitions with available resources, a persistent challenge in AI implementation.
The evaluation of process complexity is crucial in determining the suitability of AI. Processes that are most suitable for automation should be identified to avoid challenges in decision-making.
The following points should be considered when evaluating process complexity for AI suitability:
- Complexity of Processes: Some processes may be inherently complex or dynamic, making it challenging to develop AI models that can adapt and automate these tasks accurately.
- Integration with Existing Systems: Compatibility issues and integration challenges with existing systems can hinder the seamless implementation of AI automation into established workflows.
Finally, a methodological approach to assessing the current state of the organization is imperative. This includes evaluating system integration capabilities and identifying opportunities for AI intervention that align with organizational goals.
Prioritizing High-Impact Processes
In the realm of customer service, the prioritization of high-impact processes is crucial for maximizing the benefits of AI implementation. Identifying and focusing on key areas that can significantly enhance operational efficiency and customer satisfaction is essential. These processes often serve as the best candidates for AI enhancement due to their potential to streamline operations and reduce costs.
- Evaluate System Integration: Assess the integration capabilities of the systems to identify whether the AI solutions need to interface with other software or databases.
- Identify and Prioritize Opportunities: Determine which processes will benefit most from AI intervention and order them based on potential impact and alignment with organizational goals.
Balancing ambitions with available resources is a persistent challenge. It is important to consider the complexity of processes and the sophistication required for AI solutions, which can influence both development and maintenance costs. Optimal resource allocation, considering both budget and skilled personnel, is key to a successful AI strategy.
Customizing AI Solutions for Legal Tech
The customization of AI solutions in legal technology is a critical step towards ensuring that the tools are not only effective but also resonate with the specific needs of legal professionals. By tailoring AI tools to the unique challenges and complexities lawyers face, legal firms can enhance their service delivery and operational efficiency. This involves a deep understanding of the various practice areas and the development of specialized AI applications that can handle tasks ranging from document analysis to predictive case outcomes.
To achieve this, a methodical approach is necessary, starting with the identification of key areas where AI can make a significant impact. For instance, AI can be used to automate routine tasks, such as legal research or contract review, freeing up time for lawyers to focus on more complex aspects of their cases. Additionally, AI can provide advanced analytics for case prediction, helping lawyers to strategize more effectively.
The process of integrating AI into legal tech requires careful consideration of the legal firm's specific needs and the creation of bespoke solutions that can adapt to the evolving landscape of legal practice.
Ultimately, the goal is to create AI solutions that are not only technologically advanced but also practical and user-friendly for legal professionals. This requires ongoing collaboration between AI developers and legal experts to ensure that the technology is aligned with the practice's workflow and enhances the overall quality of legal services.
Financial Considerations in AI Deployment
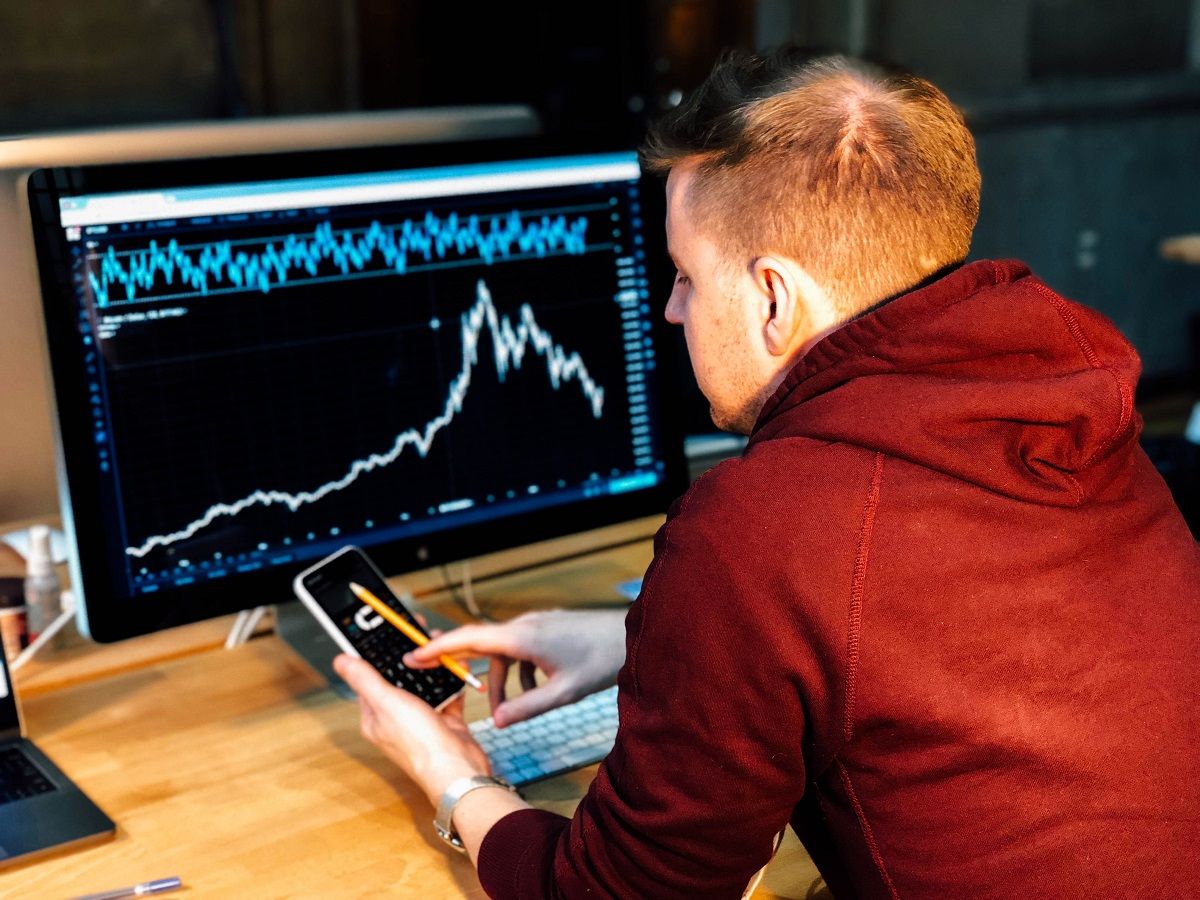
Estimating Initial and Ongoing AI Costs
Understanding the full spectrum of costs associated with AI implementation is crucial for customer service organizations. Initial expenses often encompass a variety of technological needs, from computing resources for model training to the infrastructure required for deployment. The ongoing costs, however, are not to be underestimated, as they include maintenance, updates, and potential scaling as the AI system's demands grow.
To accurately estimate these costs, a comprehensive methodology is necessary. This involves in-depth interviews to understand the specific requirements of the AI system, including computing power, storage, and data transfer needs.
The complexity of the AI solution and the sophistication required to handle intricate customer service processes can significantly affect both initial and ongoing expenses. Here's a simplified breakdown of the cost considerations:
- Initial Costs: Hardware, software, development, and integration.
- Ongoing Costs: Maintenance, updates, scaling, and technical support.
Balancing these costs with the available resources and the organization's ambitions is a delicate act that requires careful planning and realistic budgeting.
Optimizing Maintenance and Operational Expenses
Achieving operational efficiency in AI systems is pivotal for businesses aiming to streamline processes and minimize expenses. A comprehensive methodology is essential for estimating and optimizing AI maintenance costs, which includes conducting in-depth interviews to understand the specific system requirements and usage patterns of the customer.
The process of optimizing maintenance costs involves a careful balance of resources, including budget and personnel, to ensure optimal resource allocation. Scalability concerns must be addressed with a defined and balanced approach, aligning with organizational growth while also considering ethical and regulatory aspects to ensure compliance and ethical use of AI.
Customers often face initial cost concerns when implementing AI, particularly regarding infrastructure expenses. A clear understanding of the technological requirements and scalability needs is crucial for accurate cost projections and the successful deployment of AI systems.
Projecting Infrastructure Investment
When projecting infrastructure investment for AI in customer service, it's crucial to consider the technological requirements that span from high-performance computing for model training to robust deployment infrastructure. Understanding the scalability needs and computing power necessary for specific AI applications is key to accurate cost projections.
- Discuss server locations and strategic decisions between cloud-based and on-premise solutions with the technology team.
- Ensure alignment of AI infrastructure with the organization's broader technology strategy for optimal performance, security, and cost-effectiveness.
- Consider the implications of data transfer and storage on infrastructure investment.
Collaboration with the technology team is essential to navigate the complexities of infrastructure investment and to make informed decisions that balance technical requirements with financial constraints.
Aligning AI Investments with Business Value
Ensuring that AI investments translate into tangible business value is paramount for the success of any AI initiative in customer service. Define what matters for your organization with AI; this involves understanding the strategic goals and how AI can drive progress towards them. It's not just about adopting technology for the sake of innovation but about making informed decisions that align with the company's vision and objectives.
To achieve this alignment, consider the following steps:
- Conduct a thorough cost-benefit analysis to project the return on investment (ROI) for AI projects.
- Engage stakeholders across the organization to ensure that AI initiatives support broader business goals.
- Monitor and measure the performance of AI systems against predefined success metrics to ensure they deliver the expected value.
By prioritizing AI investments that are directly linked to strategic business outcomes, organizations can maximize their AI ROI and advance from experimentation to full-scale implementation.
Achieving Successful AI Integration in Legal Technology

Methodical Analysis and Targeted Interventions
The transition to AI-enhanced customer service in legal technology demands a methodical analysis of existing systems and a strategic approach to interventions. Initiating transformation requires a thorough skills assessment, pinpointing gaps, and addressing them through targeted recruitment or training. This ensures the team is equipped with the necessary AI expertise.
Before and after targeted interventions, significant changes are observed:
By systematically defining roles and fostering accountability, confusion is minimized, and a comprehensive understanding of project intricacies is ensured.
The process of analyzing systems and documents to pinpoint AI opportunities involves evaluating system integration and identifying high-impact intervention areas. Prioritizing these opportunities is crucial for aligning with organizational goals and ensuring a feasible and impactful AI implementation.
Collaborative Approach to AI Development
The integration of AI into customer service is not just a technical challenge but a collaborative endeavor. Fostering open communication and collaboration is crucial for aligning technical decisions with broader business objectives. This involves not only the technology team but also stakeholders from various departments who can provide insights into customer needs and operational workflows.
- Initiate transformation by identifying skill gaps and enhancing expertise through recruitment or training.
- Encourage collaboration to ensure a comprehensive understanding of project intricacies.
- Clearly define roles and responsibilities to minimize confusion and foster accountability.
By taking a collaborative approach, the development of AI solutions becomes a shared vision, leading to more effective and sustainable implementations.
Continuous Improvement and Adaptation
The journey of AI integration in customer service is an ongoing process that demands continuous improvement and adaptation. As customer needs and preferences evolve, so must the AI systems designed to serve them. This iterative process is crucial for maintaining relevance and effectiveness in a dynamic market environment.
- Initiate Transformation: Address limited AI expertise by enhancing skills through recruitment or training.
- Encourage Collaboration: Foster improved cross-disciplinary collaboration for a comprehensive project understanding.
- Define Roles and Responsibilities: Establish clear roles to minimize confusion and ensure accountability.
By methodically addressing each area of concern before and after intervention, organizations can create a robust framework for AI enhancement that is both resilient and adaptable to change.
Case Study: Transforming Legal Service Delivery
The transformation of legal service delivery through AI is a testament to the power of strategic and methodical integration. AI's role in enhancing legal teams is pivotal, as it streamlines the categorization and processing of incoming requests, ensuring that legal professionals can focus on more complex tasks. This case study reflects on a software development company's journey to innovate within the legal sector, particularly in assisting lawyers with litigation related to unmet legal obligations.
The project's success hinged on a multi-faceted approach that included a thorough analysis, targeted interventions, and a collaborative development process. The company emerged as a leader in legal tech innovation, ready to harness AI for improved litigation support.
The following table summarizes the key interventions and outcomes:
In conclusion, the integration of AI in legal software development was met with challenges that were overcome by strategic planning and execution. The company now stands at the forefront of legal technology, equipped to implement their AI solutions effectively.
Conclusion
In summary, the journey of integrating AI into customer service is fraught with complexities, yet it is a path that can lead to significant innovation and efficiency. Our experience with the legal tech company underscores the importance of a strategic and methodical approach to overcome challenges such as defining the scope of AI implementation, ensuring interoperability, optimizing team structures, mitigating AI risks, and integrating with existing infrastructure. By addressing these issues head-on with tailored solutions, conducting thorough analyses, and fostering collaboration, companies can not only navigate these challenges but also emerge as leaders in leveraging AI for enhanced customer service. The success of this project serves as a testament to the potential of AI in transforming service industries when implemented with foresight and precision.
Frequently Asked Questions
What are the key challenges in defining the scope for AI implementation in customer service?
Key challenges include unclear objectives and goals, ensuring data quality and availability, addressing process complexity, and balancing resources and ambitions.
How can interoperability issues with existing systems affect AI integration?
Interoperability issues can lead to compatibility problems and hinder seamless AI implementation, impacting workflow and the effectiveness of AI solutions.
What are the considerations for team restructuring during AI implementation?
Considerations include addressing the lack of AI expertise, filling data science skill gaps, and optimizing team structure for effective AI development and maintenance.
How does process complexity influence the selection of AI enhancements in customer service?
Complex or dynamic processes may require more sophisticated AI solutions, which can affect development, integration, and maintenance costs.
What financial aspects should be considered when deploying AI in customer service?
Financial considerations include estimating initial and ongoing AI costs, optimizing maintenance expenses, projecting infrastructure investments, and aligning AI with business value.
What approach was taken to ensure successful AI integration in legal technology?
A methodical analysis was conducted with comprehensive methodologies and targeted solutions, ensuring clarity, collaboration, efficiency, and continuous improvement.