The integration of Artificial Intelligence (AI) in waste management heralds a transformative approach to addressing environmental challenges. By optimizing processes such as collection, transportation, sorting, and recycling, AI not only enhances efficiency but also fosters sustainable development. This article delves into the multifaceted role of AI in revolutionizing waste management systems, from smart solutions that reduce waste generation to strategic collaborations that amplify the impact of technology on sustainability.
Key Takeaways
- AI optimizes waste management processes, including collection, sorting, and recycling, leading to improved resource efficiency.
- Machine learning and AI algorithms predict waste generation and optimize operations, contributing to significant cost and time savings.
- Smart waste management systems, powered by AI, enhance environmental sustainability by minimizing waste and maximizing resource recovery.
- Public-private partnerships are essential for implementing AI-driven waste management solutions and scaling their impact.
- AI in waste management faces challenges such as data privacy and security, but offers substantial opportunities for innovation and policy development.
Revolutionizing Waste Management with AI
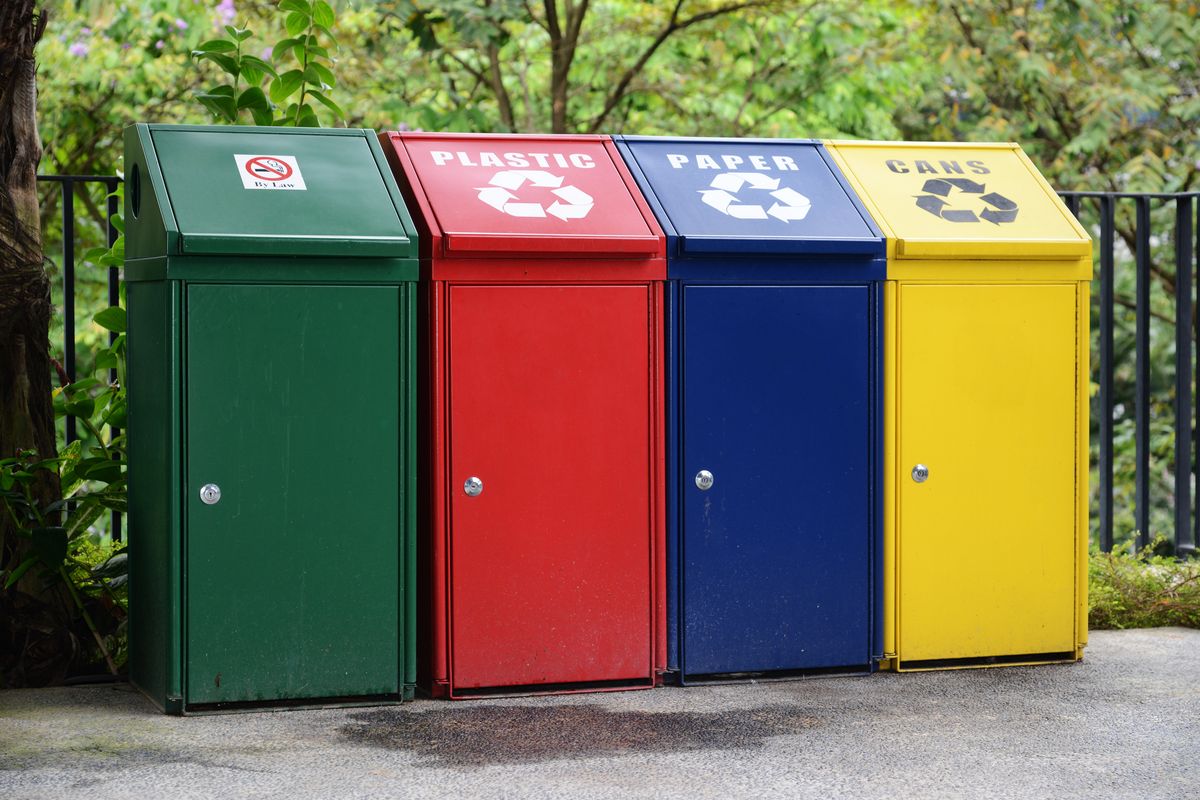
Optimizing Collection and Transportation
Integrating AI algorithms into waste management can significantly enhance the efficiency of waste collection and transportation. By optimizing the placement of dustbins and the routes for garbage collectors, not only is the process streamlined, but it also contributes to efficient resource utilization and waste minimization.
- Route optimization is a critical component, ensuring waste vehicles follow the most efficient paths, reducing fuel consumption and saving time. This approach is particularly beneficial in urban areas where traffic can significantly impact collection schedules.
Embracing circular economy principles, AI can help in creating a more sustainable waste management system by minimizing waste generation and maximizing resource efficiency.
The environmental and social benefits of such smart waste management technologies are clear. They lead to reduced environmental pollution, conservation of natural resources, and foster sustainable behavior among citizens, all while potentially reducing waste disposal costs for municipalities and businesses.
Enhancing Waste Sorting with Machine Learning
The integration of machine learning in waste management has led to the development of smart waste bins that not only classify solid waste materials but also optimize the entire collection process. These bins utilize image processing and machine learning algorithms to distinguish between recyclables and landfill waste, ensuring a more efficient sorting system.
By leveraging AI, waste management systems can now accurately identify and sort waste materials, which is crucial for both recycling efforts and waste-to-energy conversion. This technological advancement supports cost savings and process efficiency, ultimately contributing to a more sustainable environment.
The application of AI in waste sorting has transformed the traditional methods, making them more reliable and less labor-intensive.
The table below illustrates the impact of AI on waste sorting efficiency:
These improvements are not only beneficial for the environment but also for the economy, as they lead to significant reductions in operational costs.
AI-Driven Waste-to-Energy Conversion
The integration of AI into waste-to-energy systems marks a significant leap forward in sustainable waste management. AI algorithms optimize energy storage and management systems, particularly for bioenergy derived from agricultural waste. By analyzing energy demand patterns, AI ensures a more efficient conversion process, aligning energy production with consumption needs.
- Waste-to-Energy Initiatives: Leveraging AI for enhanced biogas and electricity production.
- AI in Pyrolysis: Improving carbon emission estimation and energy conversion efficiency.
- Predictive Analytics: Utilizing AI to forecast waste composition and energy potential.
AI's role in waste-to-energy conversion extends beyond mere technological advancement; it embodies a transformative approach to managing organic waste and generating renewable energy.
The potential of AI in this domain is underscored by its ability to increase the accuracy of waste characterization. For instance, Artificial Neural Networks (ANN) can predict the specific weight and composition of municipal solid waste based on socioeconomic data, leading to more informed planning and operational decisions.
AI-Powered Waste Reduction Strategies
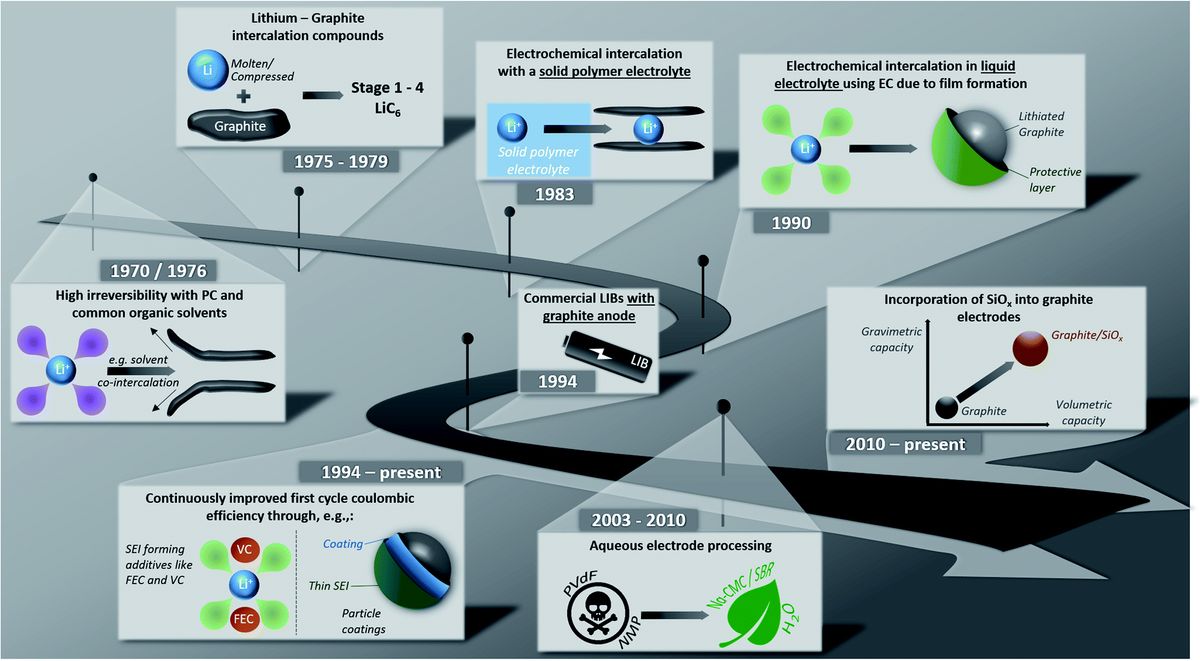
Predictive Analytics for Waste Generation
Predictive analytics is transforming the landscape of waste management by forecasting waste generation with enhanced accuracy and reliability. By integrating Artificial Intelligence (AI) and the Internet of Things (IoT), systems can anticipate the volume and types of waste, leading to more sustainable and effective management practices.
Predictive models, such as Artificial Neural Networks (ANN), utilize socioeconomic data to estimate the composition and specific weight of municipal solid waste (MSW), enabling better planning and resource allocation.
The application of AI in waste logistics not only streamlines transportation routes but also contributes to significant cost and time savings. Additionally, AI's role extends to improving waste-to-energy processes through advanced chemical analysis and optimizing pyrolysis and carbon emission estimations.
- Efficiency: AI models increase the efficiency of waste management systems.
- Cost Savings: Optimized routes and processes lead to reduced expenses.
- Sustainability: Accurate predictions help in minimizing waste generation.
- Public Health: Better waste management practices promote a healthier environment.
Smart Bins and Real-Time Monitoring
Smart bins equipped with IoT technology are transforming the landscape of waste management. These bins use sensors to monitor fill levels in real-time, enabling optimized collection routes and reducing operational inefficiencies. By integrating AI, these bins can not only classify waste materials but also predict when they will reach capacity, ensuring timely and efficient waste collection.
The integration of IoT and AI in smart bins leads to significant cost savings, enhanced process efficiency, and contributes to better public health.
Here's how smart bins contribute to smarter waste management:
- Real-time monitoring of waste levels to prevent overflow.
- Data-driven insights for forecasting waste generation patterns.
- Automated waste classification using image processing and machine learning.
- Generation of optimal collection routes for waste collectors.
The adoption of smart bin technology is a step towards a more sustainable and intelligent approach to waste management, with the potential to revolutionize how cities handle their waste.
AI in Recycling: Maximizing Resource Recovery
The integration of AI in recycling processes is a game-changer for maximizing resource recovery. AI-powered systems enhance the precision and efficiency of sorting recyclables, leading to a significant increase in the amount of materials reclaimed. For instance, robotics firm Glacier has developed AI-driven robots that streamline the recycling process, ensuring that more items are recovered and fewer end up in landfills or oceans.
By leveraging machine learning algorithms, these systems can quickly adapt to new waste streams and sorting criteria, making them invaluable in today's rapidly changing waste landscape.
The benefits of AI in recycling are not just environmental but also economic. The use of AI technologies in sorting and recovery operations can lead to cost savings and revenue generation from materials that would otherwise be discarded. Here's a snapshot of how AI contributes to the recycling industry:
- Improved sorting accuracy: AI algorithms can identify and sort waste with higher precision than manual methods.
- Increased processing speed: Automation speeds up the sorting process, allowing for more waste to be processed in less time.
- Adaptability to waste variability: Machine learning enables systems to handle diverse and changing types of recyclables.
- Data-driven decision making: Insights from AI analytics help optimize the recycling process and reduce operational costs.
Sustainable Development through AI in Waste Management

AI for Efficient Resource Utilization
The integration of AI into waste management systems is a transformative step towards sustainable development. By optimizing processes such as collection, transportation, sorting, and recycling, AI enhances the efficiency of resource use. This not only streamlines operations but also contributes to the creation of smart cities and circular economies.
AI's role in organizing essential services for the population, such as food, health care, and energy, is pivotal. It ensures that resources are used judiciously, reducing waste and promoting ecological health.
In the tourism sector, AI's demand forecasting allows for the adaptation of capacities and inventories to actual demand, preventing overbooking and wastage. Similarly, in waste management, predictive analytics can forecast waste generation patterns, leading to more informed decision-making and resource allocation.
- Optimization of waste management processes
- Creation of smart cities and circular economies
- Development of low-carbon energy systems
- Advanced guest experiences in tourism
- Demand forecasting for better resource allocation
Reducing Environmental Impact with AI
The analysis capacity of AI is pivotal in the environmental sector, enabling a deeper understanding of our ecological footprint and fostering informed decision-making. AI's role extends to preventing waste in businesses, assessing product carbon emissions, and optimizing the use of resources like energy and water. These advancements contribute to a more sustainable approach in various domains, including green building and precision agriculture.
However, the environmental cost of AI itself is a concern that could overshadow its benefits. It's crucial to align AI development with sustainability goals and to strategize for reduced energy consumption. Innovations aimed at energy efficiency and the shift towards greener energy sources are essential.
AI's transformative power in sustainability is evident. It not only enhances efficiency in waste management but also supports sustainable urban planning and smart agriculture. By leveraging big data, AI paves the way for greener solutions and the conservation of natural resources.
AI's influence on promoting responsible work behaviors and sustainable consumption is notable. It informs consumers, guiding them towards more sustainable choices, and aids in the enforcement of environmental laws. The integration of AI in environmental strategies is a step forward in achieving global sustainability targets, including those related to climate action and marine life conservation.
Public-Private Partnerships in Smart Waste Management
The collaboration between government entities, private companies, and community organizations is pivotal in advancing Smart Waste Management systems. Public-private partnerships (PPPs) are instrumental in pooling resources, expertise, and technologies to tackle the complexities of waste management. These alliances are not only about funding but also about innovation and shared responsibility.
- Government Agencies provide regulatory support and may offer financial incentives.
- Private Enterprises contribute with cutting-edge technologies and operational expertise.
- Civil Society Organizations play a role in community engagement and education.
The synergy of these diverse stakeholders under PPPs accelerates the deployment of smart technologies and sustainable practices in waste management. It ensures that the solutions developed are not only technologically advanced but also socially inclusive and environmentally sound.
The success of PPPs in Smart Waste Management hinges on clear communication, transparent objectives, and a shared vision for a sustainable future. By working together, these partnerships can lead to more efficient waste management systems that benefit all sectors of society.
Challenges and Opportunities in AI-Integrated Waste Management

Navigating Data Privacy and Security
In the realm of AI-driven waste management, data privacy and security are paramount. As systems collect and analyze vast amounts of information to optimize waste management processes, the protection of personal and corporate data becomes a critical concern.
To address these challenges, several measures can be implemented:
- Establishing robust data governance frameworks
- Ensuring compliance with global data protection regulations
- Implementing advanced cybersecurity solutions
- Conducting regular privacy impact assessments
The integration of AI in waste management necessitates a balance between innovation and the ethical handling of data. It is essential to foster trust by demonstrating a commitment to data security and privacy.
While AI offers transformative potential for waste management, navigating the complexities of data privacy and security requires a proactive and transparent approach. Stakeholders must collaborate to create an environment where data is not only leveraged for efficiency but also safeguarded against misuse.
Innovative AI Applications in Waste Logistics
The integration of Artificial Intelligence (AI) in waste logistics is a game-changer, offering significant improvements in efficiency and sustainability. AI applications in this field are diverse, ranging from optimizing transportation routes to enhancing waste-to-energy processes. By leveraging AI, waste management systems can achieve substantial cost and time savings, while also reducing environmental impact.
- Optimization of Transportation Routes: AI algorithms can analyze and predict the most efficient paths for waste collection vehicles, leading to reduced fuel consumption and lower emissions.
- Smart Waste Bins: Equipped with sensors and AI, these bins can monitor waste levels and signal when collection is needed, preventing overflow and reducing unnecessary pickups.
- Waste Sorting Robots: Utilizing machine learning, these robots can accurately identify and sort different types of waste, streamlining the recycling process.
- Predictive Waste Generation Models: AI can forecast waste production based on socioeconomic data, allowing for better resource allocation and planning.
AI's role in waste logistics not only streamlines operations but also propels us towards a more sustainable future, aligning with the circular economy model.
The potential of AI in this sector is underscored by its ability to enhance various aspects of waste management, from smart bins to waste sorting and beyond. As AI continues to evolve, its applications in waste logistics are expected to expand, further revolutionizing the industry.
The Future of AI in Waste Management Policy and Regulation
As we look to the future, the integration of AI into waste management policy and regulation holds the potential to transform the industry. Policymakers are beginning to recognize the value of AI in crafting regulations that not only protect the environment but also promote innovation and efficiency in waste management practices.
The strategic placement of AI technologies in waste management can lead to significant improvements in resource utilization and waste reduction.
The role of AI in policy-making could include the development of smart regulations that adapt to changing waste patterns and the introduction of incentives for AI-driven waste management solutions. Here's a glimpse into the possible changes:
- Development of dynamic regulations that respond to real-time data
- Incentives for businesses that adopt AI for waste optimization
- Enhanced compliance monitoring through AI systems
- Support for research into AI applications for waste reduction
These initiatives could pave the way for a more sustainable future, where waste management is not only more efficient but also more responsive to environmental needs. The challenge lies in balancing innovation with privacy, security, and ethical considerations, ensuring that the benefits of AI are harnessed responsibly.
Conclusion
The integration of Artificial Intelligence (AI) into waste management systems heralds a transformative approach to addressing environmental challenges. By optimizing collection, transportation, sorting, and recycling processes, AI not only enhances resource efficiency but also contributes to significant cost and time savings. The applications of AI in waste management—from smart bins and waste-to-energy conversion to predictive analytics and route optimization—demonstrate its potential to revolutionize the industry. As we continue to grapple with the sustainability of our planet, AI stands out as a powerful tool that can help us develop greener solutions, reduce human intervention, and protect natural resources for future generations. The synergy of AI with waste management practices is a promising step towards a more sustainable and efficient future, emphasizing the need for continued investment and innovation in this critical field.
Frequently Asked Questions
How does AI optimize waste collection and transportation?
AI optimizes waste collection and transportation by analyzing historical data to predict waste generation patterns, optimize bin placement and collection routes, and reduce transportation costs and time.
What role does machine learning play in enhancing waste sorting?
Machine learning algorithms are used to classify and sort waste materials efficiently using image processing techniques, which improves recycling rates and resource recovery.
Can AI contribute to waste-to-energy conversion processes?
Yes, AI can enhance waste-to-energy processes by predicting the composition and specific weight of waste, optimizing pyrolysis operations, and estimating energy conversion rates for better planning and efficiency.
What are smart bins and how do they use AI for waste management?
Smart bins are equipped with sensors and AI algorithms that enable real-time monitoring of waste levels, facilitating efficient collection and disposal. They can also classify waste using machine learning for better recycling outcomes.
How does AI help in reducing the environmental impact of waste?
AI helps reduce the environmental impact by improving waste sorting, reducing the need for new resources through efficient recycling, optimizing logistics to lower emissions, and enhancing waste-to-energy conversion efficiency.
What are the challenges of integrating AI into waste management systems?
Challenges include ensuring data privacy and security, managing the costs of implementing AI technologies, navigating regulatory frameworks, and fostering public-private partnerships for effective system integration.