The integration of Artificial Intelligence (AI) into agriculture is revolutionizing the industry, offering unprecedented opportunities for farm management optimization and enhanced customer support. With AI's ability to analyze vast amounts of data, farmers can now make more informed decisions, leading to increased efficiency and productivity. This article delves into the various aspects of AI application in agriculture, from precision farming and market management to livestock care and supply chain optimization, while also exploring the technological advancements that make these innovations possible.
Key Takeaways
- AI enables precision agriculture, enhancing crop yield through predictive analytics, automating operations, and monitoring soil and plant health for sustainable practices.
- Farmers can leverage AI for optimized market prediction and management, analyzing consumer demand, strategically selecting crops, and making data-driven decisions to boost profitability.
- In livestock management, AI aids in health monitoring, disease prevention, and optimizing feeding and breeding practices, contributing to better animal welfare.
- AI optimizes the agricultural supply chain, improving demand forecasting, inventory management, and reducing food waste through efficient logistics.
- Technological frameworks such as machine learning, computer vision, and natural language processing are pivotal in the successful implementation of AI in agriculture.
Precision Agriculture: Farm Optimization With AI-Powered Insights
Enhancing Crop Yield with Predictive Analytics
Predictive analytics is revolutionizing the way farmers approach crop management. By leveraging AI models that analyze historical data, weather forecasts, and current field conditions, farmers can make better-informed decisions about the entire crop lifecycle, from planting to harvesting.
Predictive analytics enables farmers to anticipate and prepare for various scenarios, reducing risks and potentially increasing both crop yield and quality.
Here are some of the key benefits of using predictive analytics in agriculture:
- Forecasting: AI models can predict weather conditions, crop failures, and pest invasions, allowing for preemptive action.
- Optimization: Suggest adjustments in farming practices to adapt to changing weather patterns and enhance soil health.
- Resource Management: Optimize irrigation and fertilization to conserve resources.
- Risk Mitigation: Early warning systems for extreme weather events protect crops and livelihoods.
Automated Operations: From Irrigation to Harvesting
The integration of AI into farm machinery is transforming the agricultural landscape. Tractors, harvesters, and other equipment are now capable of executing tasks autonomously, leading to a significant reduction in manual labor. These machines navigate fields with high precision, adapting to varying crop and soil conditions, and ensuring operations can continue 24/7.
AI-driven automation extends beyond fieldwork to include irrigation and crop monitoring systems. By analyzing data, these systems can optimize water usage, promote conservation, and minimize plant stress, contributing to a more sustainable farming practice.
Agricultural robotics are at the forefront of this revolution, with autonomous robots designed to weed, plant, and harvest. Equipped with advanced sensors and AI algorithms, they operate with unmatched precision and consistency, enhancing both productivity and crop quality.
The table below summarizes the impact of AI on key farming operations:
As AI applications in harvest automation evolve, the agricultural sector can anticipate increased productivity, sustainability, and economic benefits.
Soil and Plant Health Monitoring for Sustainable Practices
Technological innovations are revolutionizing the way farmers manage soil health, a cornerstone of sustainable agriculture. Soil sensors, satellite imagery, and soil mapping techniques provide critical insights that enable proactive management to enhance fertility and productivity. These tools help in detecting issues like nutrient deficiencies, water stress, diseases, or pest infestations early on, allowing for timely interventions that can significantly reduce potential crop loss.
The integration of AI in soil and plant health monitoring is not just about data collection; it's about transforming data into actionable insights for farmers.
AI-powered drones and satellites offer detailed images of fields, enabling the monitoring of crop health and soil quality. These images can help detect plant diseases, pests, and nutrient deficiencies at early stages, often outperforming human observation. Automated irrigation systems, which are now common in regenerative farming, use sensors for efficient water management, reducing waste and ensuring optimal growth conditions.
Sustainability is increasingly emphasized in the agriculture industry, with a growing adoption of practices that prioritize soil health, water quality, and biodiversity. Precision agriculture, employing GPS, sensors, and drones, is at the forefront of this technological shift towards eco-friendliness, offering detailed monitoring and management of soil and crop health.
Optimized Market Prediction and Management Using AI
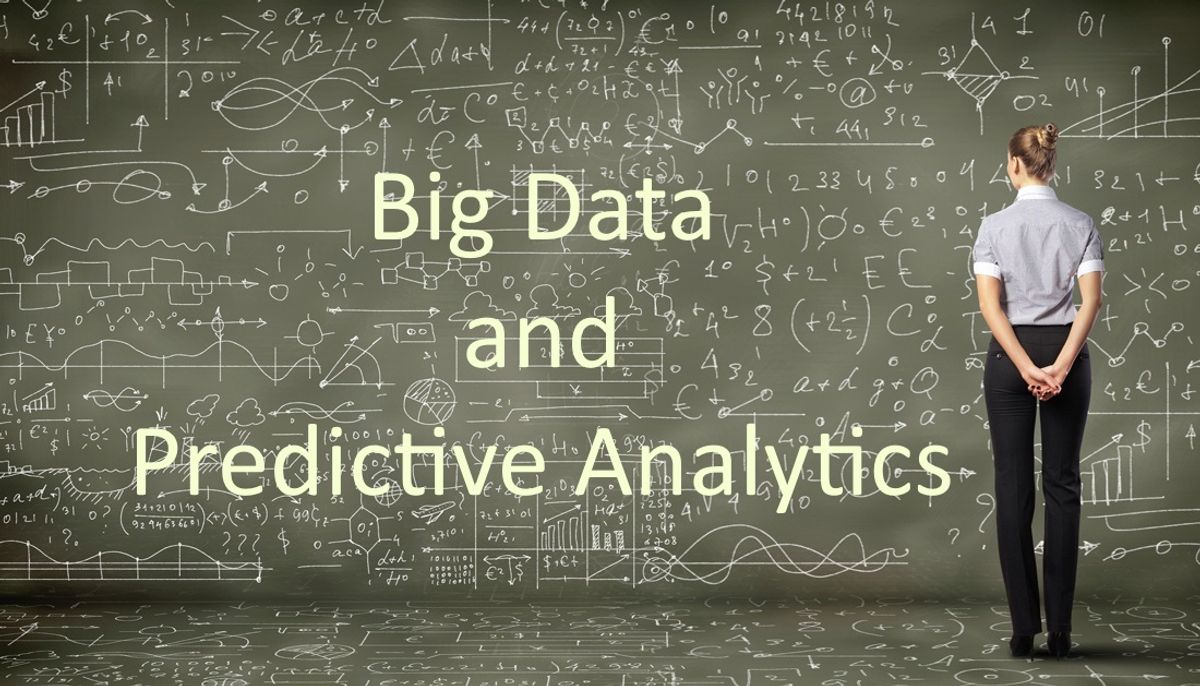
Analyzing Market Trends and Consumer Demand
In the dynamic world of agriculture, analyzing market trends and consumer demand is crucial for farmers to stay ahead. AI tools are now at the forefront of this analysis, providing insights that were once out of reach. By examining vast amounts of data, these tools can predict market shifts and consumer preferences with remarkable accuracy.
Farmers can leverage AI to make informed decisions about crop selection and harvest timing, ensuring they meet market demand efficiently.
The integration of AI in market analysis allows for a nuanced understanding of the agricultural landscape. For instance, predictive analytics can forecast demand for specific crops, helping farmers to optimize their planting schedules and reduce the risk of overproduction.
Here's how AI contributes to market trend analysis:
- Predictive Analytics: Forecasting future market conditions and consumer behavior.
- Data Collection: Gathering consistent data from various sources for comprehensive analysis.
- Precision Agriculture Tools: Utilizing advanced technologies to align farming practices with market needs.
Strategic Crop Selection and Timing for Market Success
The integration of AI in agriculture has led to a revolution in strategic crop selection and timing, ensuring that farmers' decisions are data-driven and aligned with market demands. By analyzing vast amounts of data, AI helps in forecasting seasonal weather conditions, which is crucial for determining the best planting and harvesting times. This predictive capability is essential for mitigating risks associated with adverse weather and optimizing agricultural outputs.
- Forecasting seasonal weather conditions
- Adapting planting schedules
- Choosing resilient crop varieties
The ability to adapt to shifting climatic trends and market demands is a cornerstone of modern agriculture. AI's predictive analytics provide a framework for resilience and sustainability in farming practices.
AI tools also enable farmers to analyze market trends and consumer demand, which guides them in selecting the most profitable crops. The strategic use of AI in agriculture not only enhances farm profitability but also promotes sustainable practices that are vital for feeding a growing global population.
AI-Driven Decision Making for Farm Profitability
The integration of AI-driven decision-making tools is revolutionizing the agricultural industry, offering unprecedented precision in managing farm operations for enhanced profitability. These tools analyze vast amounts of data to inform strategic decisions, from crop selection to the timing of market entry.
- AI applications assess market trends and consumer demand, guiding farmers on what and when to plant.
- They enable the optimization of resource use, reducing costs and increasing efficiency.
- By eliminating market asymmetries, AI empowers farmers to engage more competitively in regional and global markets.
AI's success in agriculture is not just about technological advancement; it's about creating a sustainable ecosystem that supports farmers and optimizes the entire value chain.
Accessible via Wi-Fi and customizable to various farming scenarios, AI applications ensure that every aspect of farm management, from irrigation to yield management, is optimized. This leads to streamlined operations, cost savings, and ultimately, a significant boost in farm profitability.
AI Facilitates Enhanced Livestock Management
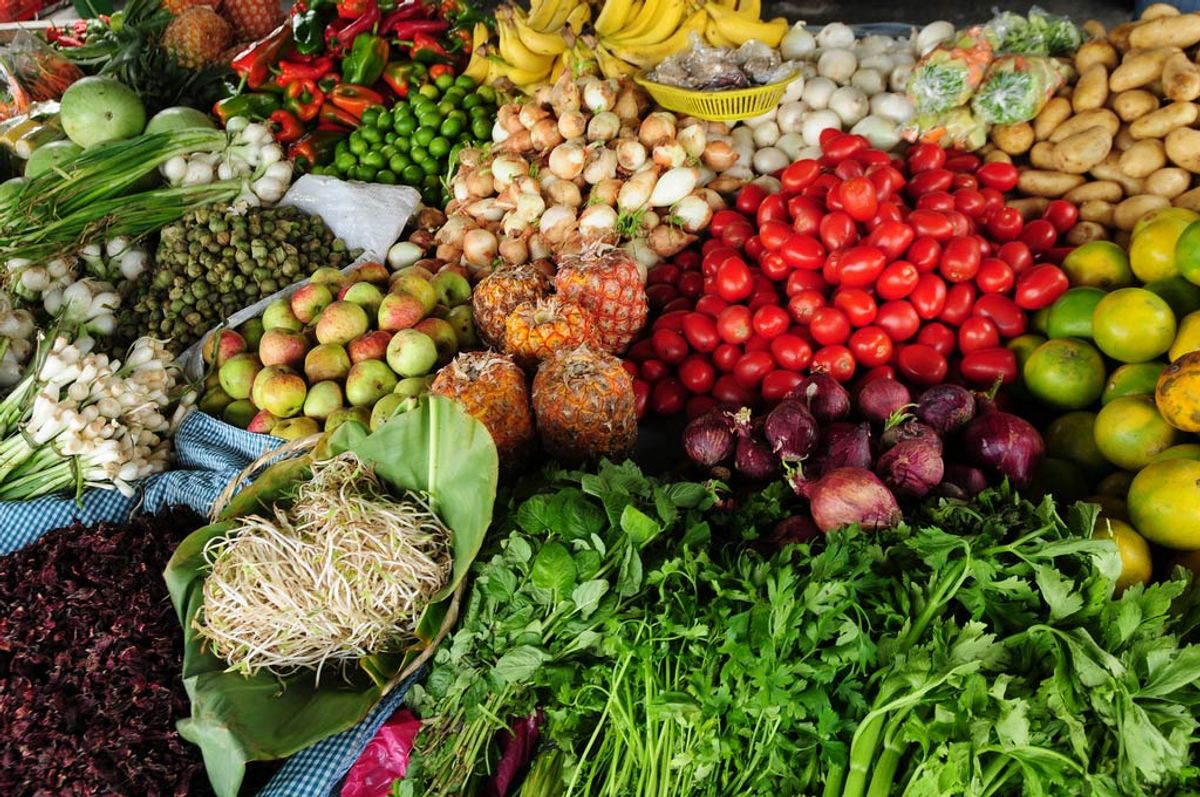
Health Monitoring and Disease Prevention with AI
The integration of AI in livestock management is revolutionizing the way farmers monitor the health and well-being of their animals. Real-time monitoring and predictive analytics work in tandem to provide farmers with early warnings of health issues, allowing for prompt and precise interventions. This not only ensures the health of the livestock but also enhances overall farm productivity.
By employing sensors and cameras, AI systems can track vital signs and behaviors, alerting farmers to any abnormalities. This proactive approach to health monitoring is crucial in preventing diseases and reducing the risk of large-scale outbreaks. The potential application of artificial intelligence in veterinary clinical settings is vast, with AI facilitating the management of complex subjects like predictive epidemiology.
AI-driven health monitoring systems are essential for maintaining high standards of animal welfare and can significantly reduce the reliance on antibiotics through early detection and treatment of illnesses.
The table below outlines the key benefits of using AI for health monitoring and disease prevention in livestock management:
Optimizing Livestock Feeding and Breeding Practices
The advent of AgriTech solutions has revolutionized the approach to precision livestock farming, where the focus is on catering to the individual needs of each animal. Automated feeding systems are now capable of delivering tailored diets that meet the specific nutritional requirements of livestock, ensuring optimal growth and health.
By integrating advanced technologies, farmers are now able to monitor the health and behavior of their animals with unprecedented precision, leading to enhanced animal welfare and productivity.
In the realm of breeding, AgriTech has introduced tools such as artificial insemination and genetic testing, which aid in achieving superior breeding outcomes and livestock quality. These innovations not only improve the efficiency of farm operations but also contribute to sustainable agricultural practices.
The table below summarizes the key benefits of utilizing AI in livestock management:
Innovative Surveillance Systems for Animal Welfare
The advent of AI in agriculture has led to the development of innovative surveillance systems that significantly enhance animal welfare. By employing sensors and wearable devices, farmers can now collect real-time data on various parameters such as activity levels, body temperature, and feeding patterns. This wealth of information is crucial for detecting early signs of illness or distress, enabling prompt intervention and ensuring the well-being of the livestock.
The integration of precision farming technology and animal-based data has revolutionized the way farmers approach animal welfare.
Furthermore, these systems have paved the way for precision livestock farming practices, where the needs of individual animals are meticulously monitored and managed. Automated feeding systems, for example, deliver customized diets to each animal based on their nutritional requirements, while health monitoring systems detect signs of disease or infection early on.
- Automated Feeding Systems: Deliver customized diets based on nutritional needs.
- Health Monitoring Systems: Early detection of disease or infection.
- Activity and Temperature Monitoring: Real-time data collection for prompt care.
Optimize Supply Chain from Farm to Table

Demand Forecasting and Inventory Management
The integration of AI into agricultural supply chains has revolutionized the way agribusinesses forecast demand and manage inventory. By leveraging machine learning algorithms, companies can anticipate the market needs for agricultural products with remarkable accuracy. This not only ensures that inventory levels are kept optimal, reducing waste, but also guarantees that products reach the market at their peak demand.
- Predictive analytics enable the adaptation of planting schedules and crop selections to forecasted seasonal weather conditions, such as droughts or heavy rainfall.
- Real-time data analysis optimizes delivery routes and schedules, taking into account traffic, weather, and delivery windows.
- Strategic inventory management is facilitated by AI, which can reduce transportation costs and enhance delivery times.
By utilizing AI for demand forecasting and inventory management, agribusinesses can achieve a more efficient and responsive supply chain, from farm to table.
The table below outlines key components of AI-driven logistics in agriculture:
Efficient Route Planning and Delivery Scheduling
In the realm of agriculture, efficient route planning and delivery scheduling are pivotal for ensuring that fresh produce reaches the market in prime condition. AI systems are adept at analyzing multiple variables such as real-time traffic data, weather conditions, and delivery deadlines to devise the most effective transportation strategies. This not only reduces transportation costs but also significantly improves delivery times.
- Resource allocation and scheduling are critical components in the logistics of farm produce.
- An integrated vehicle routing model takes into account the perishability of goods and market prices, optimizing the distribution of fresh agricultural produce.
By leveraging AI for route optimization, agribusinesses can achieve a harmonious balance between supply chain efficiency and customer satisfaction.
Reducing Food Waste through AI-Enhanced Logistics
The integration of AI into agricultural logistics has brought about a significant reduction in food waste. AI-driven inventory systems are pivotal in optimizing stock levels and ensuring the timely distribution of perishable goods. By analyzing various factors such as market demand, perishability, and delivery deadlines, these systems facilitate better planning and supply chain efficiency.
- Predictive analytics for precise demand forecasting
- Real-time monitoring of stock levels and environmental conditions
- Optimized delivery routes based on traffic and weather data
AI not only enhances the efficiency of the supply chain but also contributes to sustainability by minimizing waste. This is achieved through smarter inventory management and logistics planning that adapt to the dynamic nature of agricultural products.
The use of AI in managing the supply chain from farm to table ensures that products reach the market in the most efficient way possible. It takes into account real-time data to predict demand, manage inventory, and plan logistics, thus fast-tracking the entire process from production to consumer.
The Tech Framework: Explore Which Technologies Help AI Succeed
Machine Learning and Deep Learning in Agriculture
At the forefront of agricultural innovation, machine learning and deep learning technologies are revolutionizing the industry. These AI techniques are instrumental in enhancing various aspects of farming, from crop monitoring to yield prediction. Machine learning algorithms are adept at analyzing vast datasets to forecast crop yields, detect diseases, and manage pests, while deep learning excels in image-based monitoring and computer vision tasks such as weed detection.
The integration of machine learning and deep learning in agriculture is not just about technological advancement; it's about creating a sustainable and efficient future for farming.
Deep learning, in particular, has shown great potential for the agriculture industry, offering modern techniques for accurate image processing and data analysis. This has led to the deployment of AI in a range of tasks, including precision farming and the operation of autonomous farming equipment. However, the journey of integrating these technologies comes with its own set of challenges and opportunities, paving the way for future directions in agricultural AI.
Computer Vision for Crop and Livestock Monitoring
The integration of computer vision into agriculture has revolutionized the way farmers monitor crop and livestock health. By utilizing drones and field-installed cameras, image-recognition algorithms analyze photos to detect early signs of disease, stress, and nutrient deficiencies. This proactive approach allows for timely interventions, reducing the reliance on chemical treatments and supporting sustainable farming practices.
- Early detection of weed infestations and nutritional deficits
- Precise application of pesticides and fertilizers
- Forecasting crop yields with AI models
The synergy between AI and imaging technology not only enhances the efficiency of crop management but also contributes to the overall health of the ecosystem by minimizing environmental impact.
Furthermore, computer vision aids in livestock management by monitoring animal behavior and health indicators, leading to improved welfare and productivity. The potential of AI in agriculture is vast, with ongoing research expanding the capabilities of these technologies to meet the challenges of modern farming.
Natural Language Processing for Enhanced Customer Support
In the realm of agriculture, Natural Language Processing (NLP) stands out as a transformative technology. By analyzing vast amounts of unstructured data, such as customer inquiries, feedback, and social media interactions, NLP enables a more nuanced understanding of client needs and preferences. This, in turn, allows for the development of smarter, more responsive customer support systems.
The application of NLP in agriculture extends beyond customer interaction. It facilitates knowledge management by extracting relevant information from a diverse data landscape. This can aid farmers in accessing valuable insights, which are crucial for making informed decisions. For instance, NLP can interpret weather reports, research papers, and market trends, translating them into actionable advice for farmers.
The integration of NLP with other AI technologies has the potential to revolutionize the agricultural sector, offering a level of precision and personalization previously unattainable.
Here's a glimpse of how NLP is being utilized in the agricultural sector:
- Customer Query Resolution: Automating responses to frequently asked questions and providing personalized advice.
- Sentiment Analysis: Gauging public sentiment about agricultural products and practices.
- Language Translation: Breaking down language barriers to disseminate knowledge globally.
- Voice-Activated Systems: Enabling hands-free operation for farmers through voice commands.
Conclusion
The integration of AI in agriculture is revolutionizing the industry, offering unprecedented opportunities for farm management optimization and enhanced customer support. From precision agriculture to supply chain efficiency, AI-powered tools are enabling farmers to make data-driven decisions, improve resource utilization, and increase profitability. Despite the challenges such as data privacy, scalability, and accessibility, the potential benefits of AI in agriculture are immense. As technology continues to advance, it is imperative for the agricultural sector to embrace AI to remain competitive and sustainable. The future of farming is intelligent, and AI is at the forefront of this transformation, promising a more efficient, productive, and environmentally friendly agricultural landscape.
Frequently Asked Questions
How does AI contribute to precision agriculture and farm optimization?
AI enhances precision agriculture by providing insights through predictive analytics, automating operations from irrigation to harvesting, and monitoring soil and plant health for sustainable practices. It helps in maximizing output while preserving resources, and streamlining processes like picking, sorting, and packaging to improve efficiency and profitability.
In what ways can AI optimize market prediction and management for farmers?
AI tools analyze market trends, commodity prices, and consumer demand to assist farmers in making informed decisions about crop selection and optimal selling times. This leads to increased profitability by eliminating market asymmetries and allowing farmers to participate more effectively in regional and global value chains.
How is AI used in livestock management?
AI and machine learning techniques are employed in livestock management to monitor health and well-being, improve feeding practices, detect illnesses early, and optimize breeding. Sensors and cameras monitor vital signs and behaviors, providing alerts to potential issues before they escalate.
What role does AI play in optimizing the agricultural supply chain?
AI aids in demand forecasting, inventory management, and optimizing delivery routes to reduce food waste and enhance logistics. It helps to determine the most efficient transportation routes and schedules, taking into account factors such as perishability, market prices, and delivery deadlines.
What challenges does AI face in the agricultural sector?
Despite its potential, AI in agriculture faces challenges like data privacy concerns, scalability issues, and accessibility for smallholder farmers. Addressing these challenges is crucial for the widespread adoption and effectiveness of AI technologies in the sector.
What technologies underpin the success of AI in agriculture?
Technologies such as machine learning, deep learning, computer vision, and natural language processing underpin the success of AI in agriculture. They are used for tasks like crop monitoring, disease detection, yield prediction, precision farming, and autonomous equipment operation.